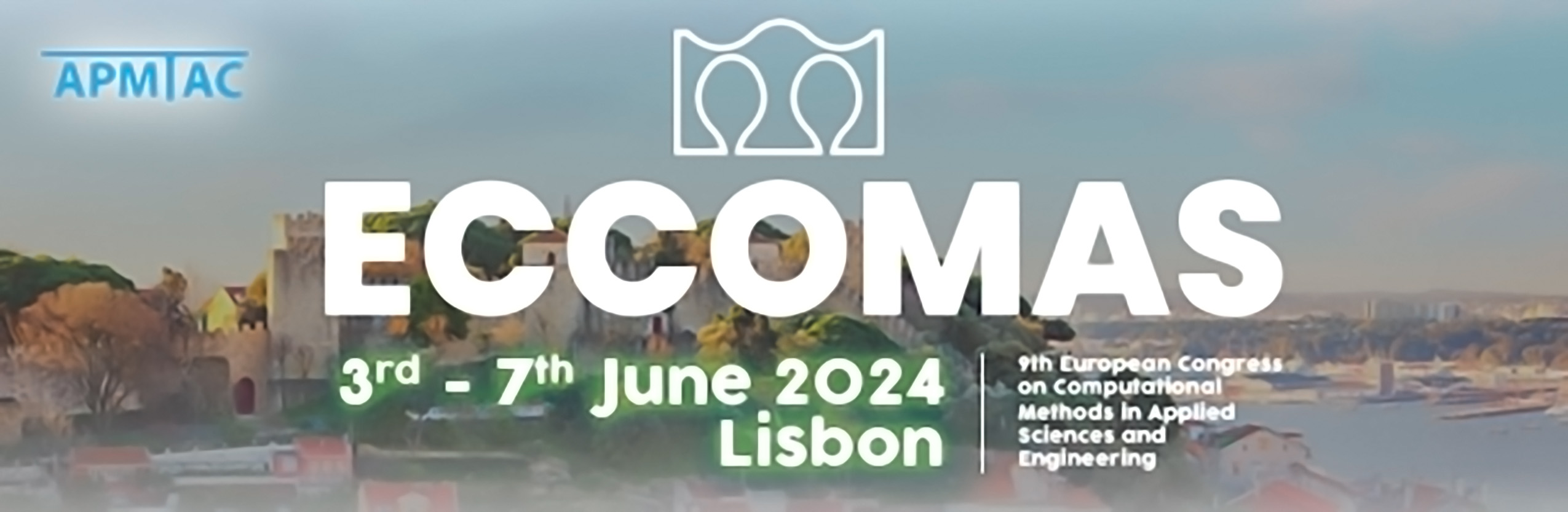
Multiscale modeling with physics-augmented neural networks
Please login to view abstract download link
The formulation and calibration of constitutive models is still a challenging task for materials which exhibit complex nonlinear behavior. Due to this, data-driven methods have become increasingly popular recently. Thereby, a promising field of application is the usage of such methods for the acceleration of multiscale simulations [1]. Herein, we present an approach based on physics-augmented neural networks (PANNs) [2,3] that are applied as macroscopic surrogate models. PANNs are designed to fulfill as many conditions as possible from constitutive modeling by construction and are very flexible at the same time. Our approach allows the efficient simulation of materials with complex underlying microstructures which reveal an overall anisotropic and nonlinear behavior on the macroscale. Thereby, we restrict ourselves to finite strain hyperelasticity problems in the first part of the talk. By using a set of problem-specific invariants as the input of the PANN and the Helmholtz free energy density as the output, several physical principles, e.g., objectivity, material symmetry or thermodynamic consistency are fulfilled a priori [2, 3]. The invariants are formed from structure tensors and the right Cauchy Green deformation tensor. Necessary data for the training of the PANN surrogate model are collected via computational homogenization of representative volume elements (RVEs). Besides the network parameters, the structure tensors are automatically calibrated during training so that the underlying anisotropy of the RVE is reproduced in the best possible way. The developed approach is exemplarily applied to several descriptive examples. Finally, an extension of the approach to magneto-hyperelasticity is shown [3]. REFERENCES [1] K. A. Kalina, L. Linden, J. Brummund, M. K¨astner, FEANN: an efficient data-driven multiscale approach based on physics-constrained neural networks and automated data mining. Comput. Mech., Vol. 71, 2023. [2] L. Linden, D. K. Klein, K. A. Kalina, J. Brummund, O. Weeger, M. K¨astner, Neural networks meet hyperelasticity: A guide to enforcing physics. J. Mech. Phys. Solids, Vol. 179, 2023. [3] K. A. Kalina, P. Gebhart, J. Brummund, L. Linden, W. Sun, M. K¨astner, Neural network-based multiscale modeling of finite strain magneto-elasticity with relaxed convexity criteria. arXiv Preprint, 2023.