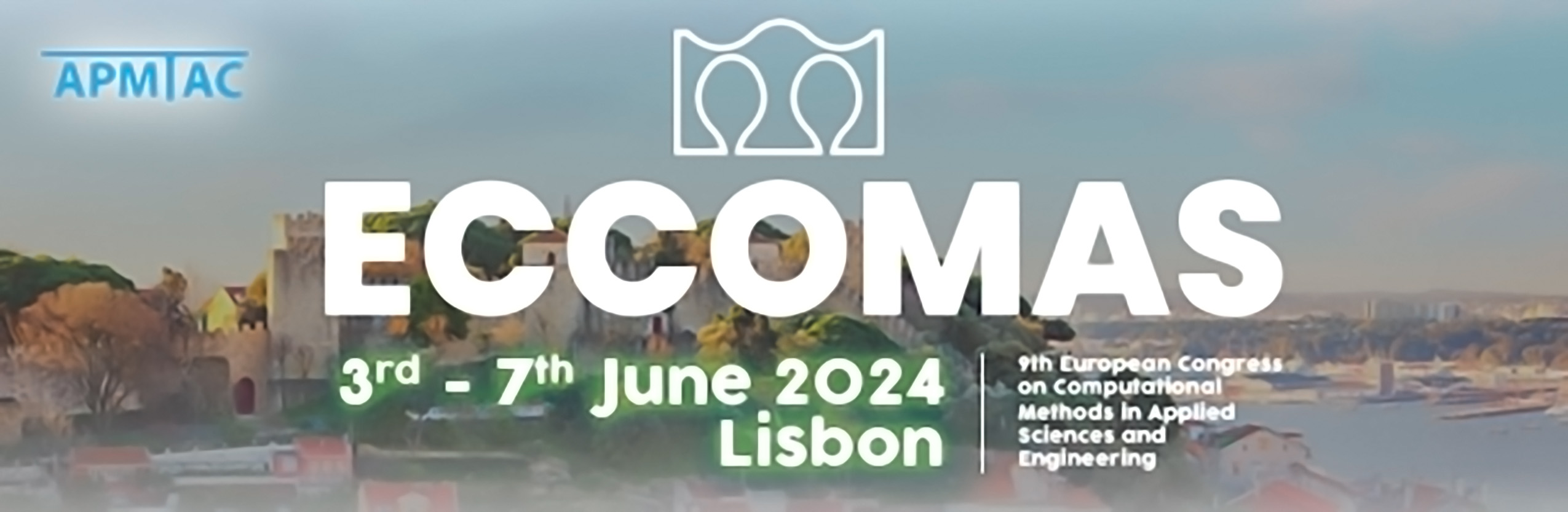
Machine Learning (ML) Application for the Investigation of Nucleation Boiling in Pressurised Water Reactor (PWR).
Please login to view abstract download link
Nucleation boiling in heat transfer system, such as nuclear reactor, plays a critical role in the overall thermal performance and safety of systems. Understanding the early stage dynamics of nucleation boiling, is essential in preventing potential safety hazards, such as post critical heat flux (CHF) and loss of coolant accident (LOCA) events. As a two-phase flow phenomenon, early stage of nucleation boiling is usually investigated with the study of the dynamics of single rising bubble (SRB). Due to flow parameter instabilities at vapor-liquid interface for rising bubble as a result of phase change involved in the process, it is crucial to incorporate ML algorithm to SRB models for accurate evaluation of the flow parameters. This study focuses on the application of a machine learning algorithm to investigate the intricate details of the SRB phenomenon during the early stages of nucleation boiling in PWR. The research employs high-order accurate CFD models coupled with compressive advection interface capturing model (CAICM) to investigate the dynamics (onset formation, growth and flow) of SRB under reactor conditions. Applying ML techniques, the study aims to unravel complex relationships within the vast datasets generated during these simulations, by ensuring accurate flow parameter evaluation during phase changes. By incorporating ML algorithms into higher-order CFD models, the study seeks to identify key parameters influencing bubble dynamics, including size, velocity, and shape deformation. The results of this investigation are anticipated to give significant contributions to the development of more reliable predictive models for nucleation boiling in PWRs. In addition to enhancement of the efficiency of data interpretation, incorporation ML into the models enables the detection of correct trends and dependencies of parameters which could be difficult to determine using traditional analytical approaches. Consequently, the findings of this research hold the potential to inform improved thermal management strategies, accident prediction, and safety improvements in PWRs, thereby enhancing the overall reliability and sustainability of nuclear energy production.