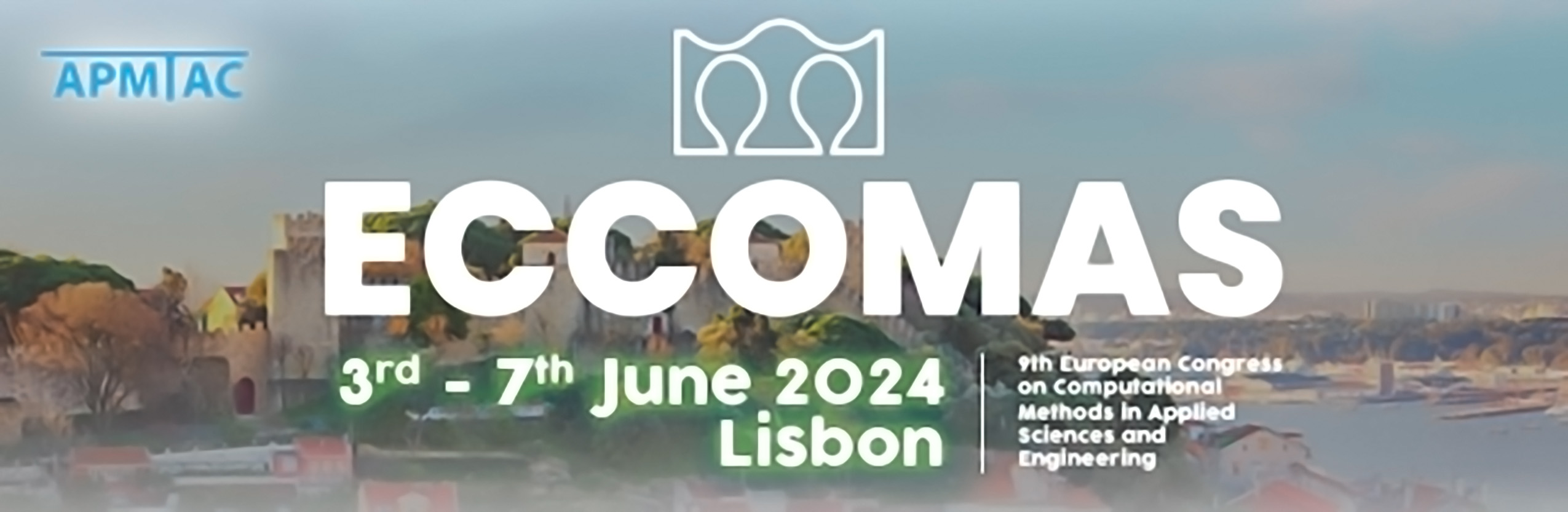
On the use of bifurcation curves for system identification and model updating purposes
Please login to view abstract download link
The ever-increasing demand for highly performant mechanical systems often leads them to exhibit nonlinear behaviours. Furthermore, in recent years, a significant amount of research was devoted to investigating how nonlinearities can be exploited for increased performance. In order to achieve better designs and to ensure the performance, safety and resilience of mechanical systems throughout their service life, it is of utmost importance to have access to nonlinear models with both qualitative and quantitative predictive capabilities. Due to potentially strong nonlinear effects, traditional system identification features based on linear modes often prove suboptimal. Nonlinear normal modes have emerged as a key feature for identifying and updating nonlinear models due to their capability of capturing the frequency/energy dependence of nonlinear systems. Nevertheless, several other nonlinear phenomena can be observed in practice. Amongst the salient features distinguishing nonlinear systems from their linear counterparts, bifurcations occupy a prominent position, as they act as local organizing centres for the system's dynamics. Bifurcation curves define the limits of stability of nonlinear systems and regions of multi-stability. Consequently, they provide both quantitative and qualitative information on systems operating in strongly nonlinear regimes. In this work, we show that bifurcation curves can be utilized as features to carry out nonlinear system identification and model updating. Our methodology is based on minimizing the distance between experimental bifurcation curves evaluated through control-based continuation~\cite{rensoncbc} and numerical ones computed with bifurcation tracking. A nonlinear energy harvester model is employed to demonstrate the methodology's performance in identifying nonlinear models within strongly nonlinear regimes across a broad range of operating conditions.