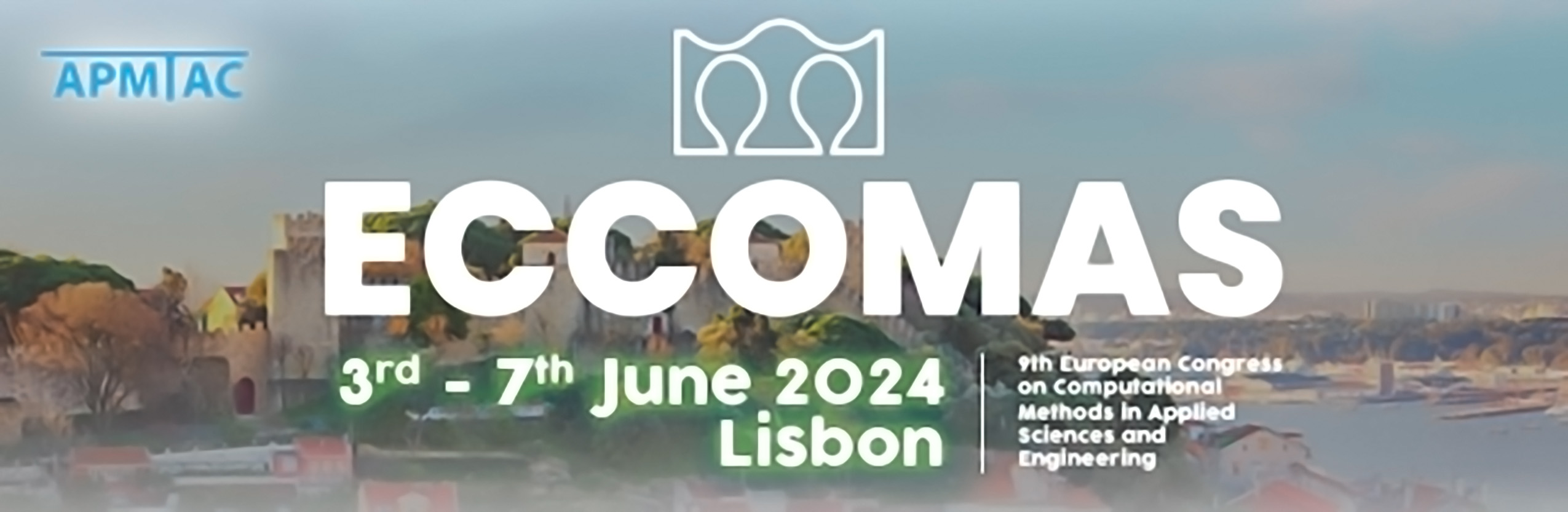
Optimal Transport-Inspired Model Order Reduction: Leveraging Wasserstein-Based Kernel and Shifted POD
Please login to view abstract download link
Reduced order modeling has emerged as a powerful technique to speed up the investigation of complex systems described by partial differential equations (PDEs). Traditional linear methods, such as proper orthogonal decomposition (POD), have limitations in capturing complex nonlinear phenomena and suffer from information loss. Nonlinear reduction techniques, including deep learning (DL) frameworks, have gained popularity for addressing these challenges. This research introduces an innovative Reduced Order Model (ROM) framework, where we leverage the power of Optimal Transport (OT) theory in combination with two distinct dimensionality reduction techniques: Kernel Proper Orthogonal Decomposition (kPOD) and shifted Proper Orthogonal Decomposition (sPOD). The OT-driven Wasserstein distance-based kernel captures intrinsic data features, while kPOD and sPOD provide effective nonlinear reduction strategies. To effectively use kPOD, we address the challenge of approximating the backward mapping from the latent space to the full-order solution. We employ an autoencoder architecture, where the encoding layer learns the reduced representation. Training utilizes the Sinkhorn algorithm, a computationally efficient method for solving the Optimal Transport (OT) problem. Expanding our focus, we explore atmospheric mesoscale benchmarks. Here, we introduce a novel approach using sPOD based on the optimal transport map. Applied to simulations of a dry atmosphere, our method holds promise for enhancing model order reduction techniques in atmospheric dynamics.