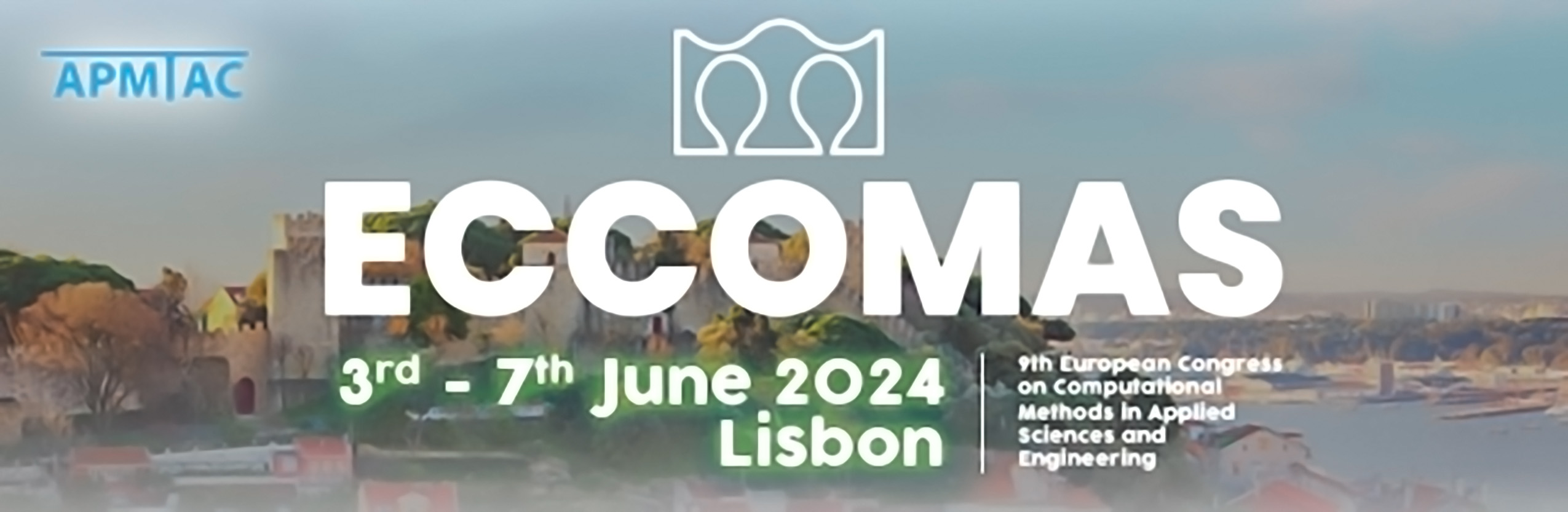
Ensemble Kalman Filtering for Stochastic Phase-field Models of Brittle Fracture
Please login to view abstract download link
Phase-field modelling of brittle fracture has received a lot of attention in the computational mechanics community. The phase-field approach provides an elegant way to compute the growth of cracks in an elastic solid in a regularised manner, thus circumventing the problem of discontinuities in the solution and providing mesh-independent results. Nevertheless, the model is subject to uncertainty in material parameters, initial conditions et cetera. This uncertainty leads, in turn, to an uncertainty in the crack path: Different parameter realisations yield different paths. Besides uncertainty in the parameters, model error occurs due to unresolved physics, erroneous modelling assumptions or inadequate discretisation. The ability to continuously monitor modern structures using sensors makes it possible to complement modelling predictions with e.g. displacement measurements. In this contribution, we connect these two complementary sources of information and present a Bayesian inference procedure which allows to update the current state of the model prediction with incoming sensor data. We construct a Bayesian prior for the model state (both displacements and phase-field) and employ an ensemble Kalman filter (EnKF) to perform the update of the state. Prior to each update, the hyperparameters of a Gaussian Process data model, adopted from the statistical FEM approach, are estimated from data in order to find an accurate estimate of the model error. In the ensemble Kalman filter, the update is computed by performing a so-called Kalman shift on each ensemble member. We demonstrate that the updated state converges to the ground truth. As opposed to traditional Bayesian inversion techniques, not the model parameters but the model state, i.e. both the displacement vector field and the phase field, is subjected to Bayesian inference. It is worth nothing that only displacements are observed, yet, due to the strong correlation between both fields, also the posterior phase-field is inferred. Different numerical examples and benchmarks are given to illustrate the performance and accuracy of the proposed method.