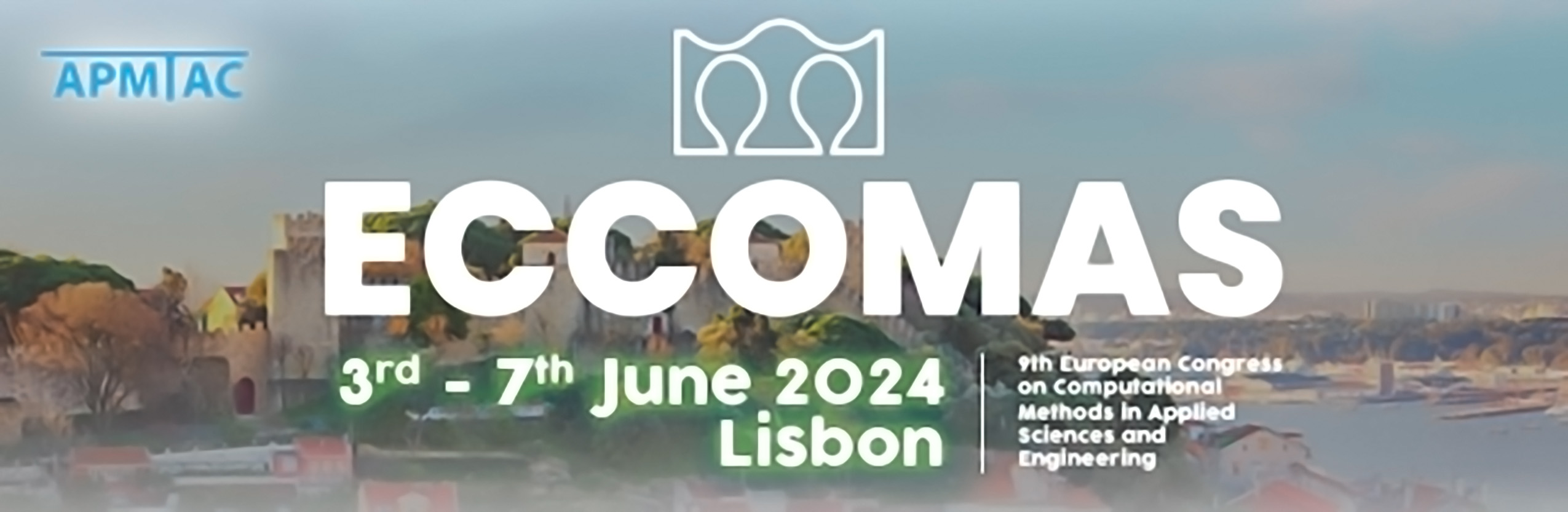
Data-driven methods in energy transition
Please login to view abstract download link
In our presentation, we'll explore using Reproducible Kernel Hilbert Space (RKHS) as a machine-learning tool for probability forecasting amidst energy transition challenges. We'll highlight how kernel methods, coupled with multiresolution analysis, apply in real cases like NASA's PRS2022, RANS-Airfoils, and climate forecasts. These examples will help understand reactive flow in alternative fuel combustion scenarios. Later, we'll focus on embedding distributions, especially kernel mean embedding. We'll compare kernel herding and Nystrom based approximations for pricing density estimation within the Swiss energy market, offering insights into real market scenarios. The goal is to develop data-centric learning to tackle the complexities of energy transition.