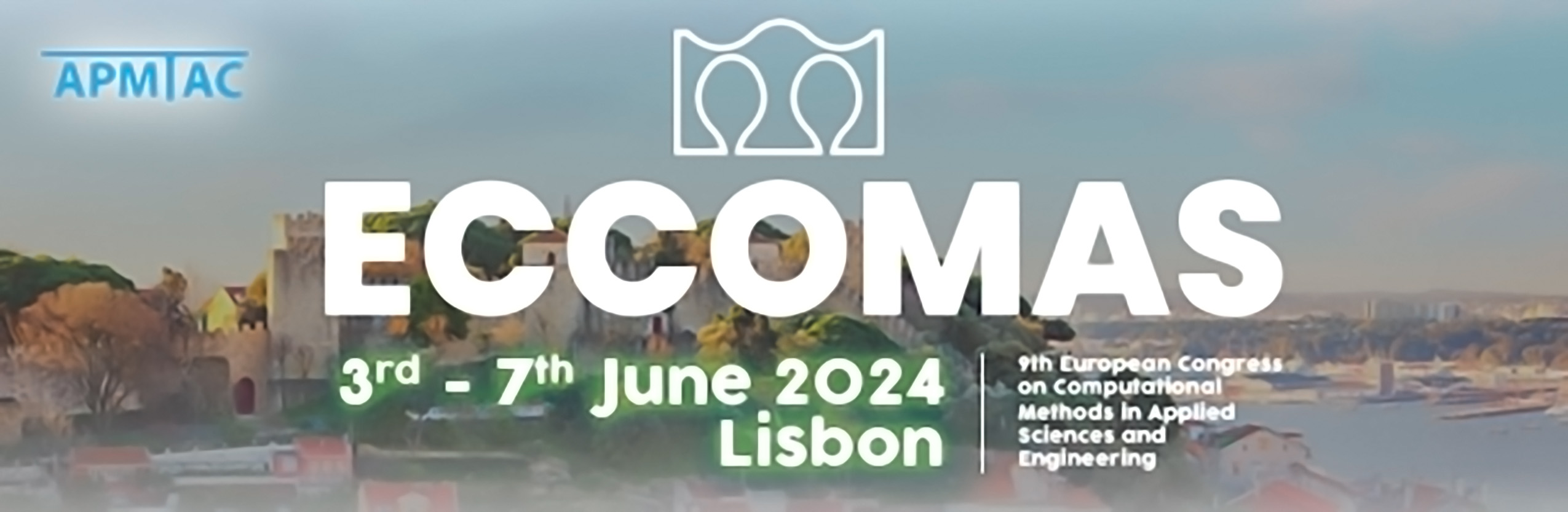
A Parametric Investigation of the Train-Test Ratio for Machine Learning Algorithms in Structural Mechanics Applications
Please login to view abstract download link
This research investigates the impact of varying train-test ratios on machine learning (ML) algorithms in the context of structural mechanics. Notably, it reveals that these algorithms favour lower train-test ratios to counter overfitting and enhance robustness. The study employed five datasets with various characteristics, objectively evaluating the train-test ratios' influence when implementing the under-study ML algorithms. This research identified optimal ratios for different ML algorithms, contributing to a more tailored approach to ML algorithmic performance assessment. According to the parametric investigation, it was found that the range of train-test ratios that is optimal for most algorithms is 0.1-0.15, suggesting a need to explore a wider range of ratios with smaller intervals for a comprehensive and more detailed performance assessment. Finally, the study calls for future research to explore the response of ML algorithms when trained on numerical versus experimental datasets, potentially leading to a generalised recommendation when dealing with structural mechanics applications.