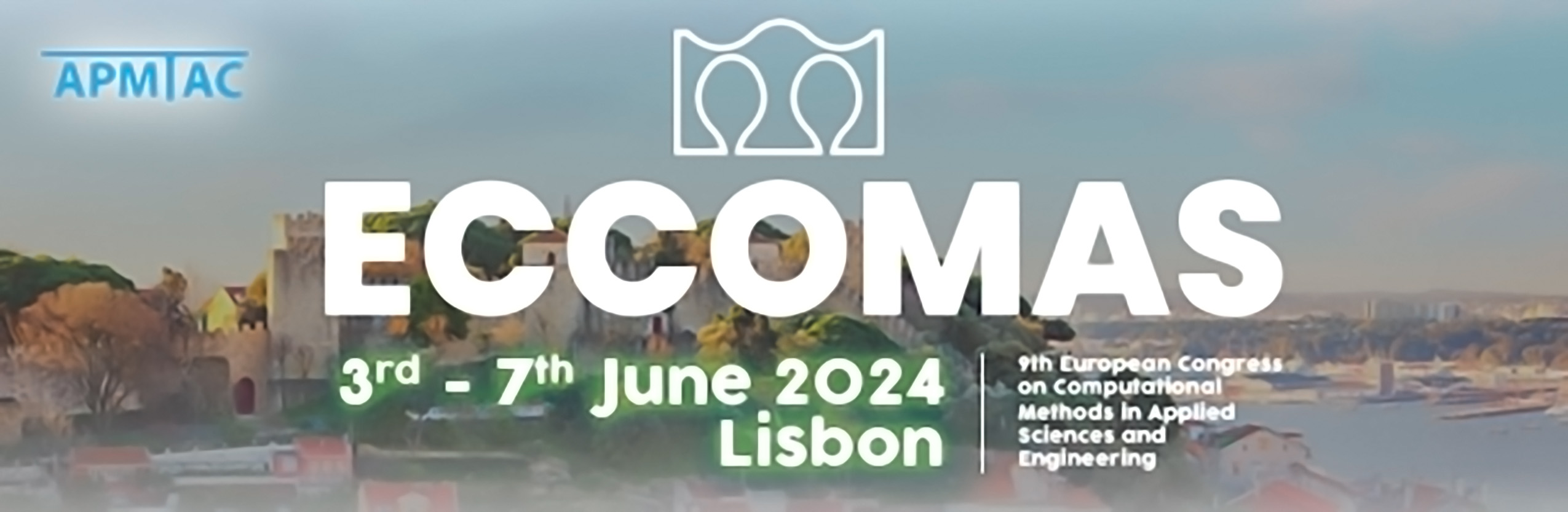
Convolutional Neural Network Coupled with a Constrained CP-decomposition Model for Predicting Upscaled Permeability
Please login to view abstract download link
Permeability is an essential parameter in understanding fluid flow behaviours in subsurface reservoirs. The prediction of subsurface fluid flows requires a detailed reservoir model capturing the multiple length scales of heterogeneities associated with geological properties. A significant challenge of porous media multiphase flows is the appropriate representation of geophysical properties (e.g., permeability, porosity, etc.) in heterogeneous reservoirs across multiple length scales. The challenges considered here are two-fold. First, obtaining detailed permeability distribution in a field-scale geological domain is impossible. Secondly, the large-scale data set involved with running the most realistic and accurate simulations is computationally expensive. These challenges gave birth to upscaling, a model order reduction method. In traditional upscaling models, geological properties (of- ten represented as scalars) are homogenised across length scales with reasonable accuracy. More sophisticated upscaling models based on tensor-space projections were proposed to represent high-fidelity permeability data with a reduced-order (low-rank) tensor representation with good accuracy. This work evaluates how a machine learning algorithm can predict upscaled permeability for geothermal reservoirs. We used convolutional neural networks (CNNs) to train a set of permeability realisations. Here, the convolutional layers are represented in a decomposed form by incorporating a constrained CP decomposition to reduce the parameter in the convolutional layer. The prediction performance of our CNN is examined, where numerical simulations demonstrate that the predicted upscaled permeability with low mesh resolution matches results from high mesh resolution and large-scale data with good accuracy.