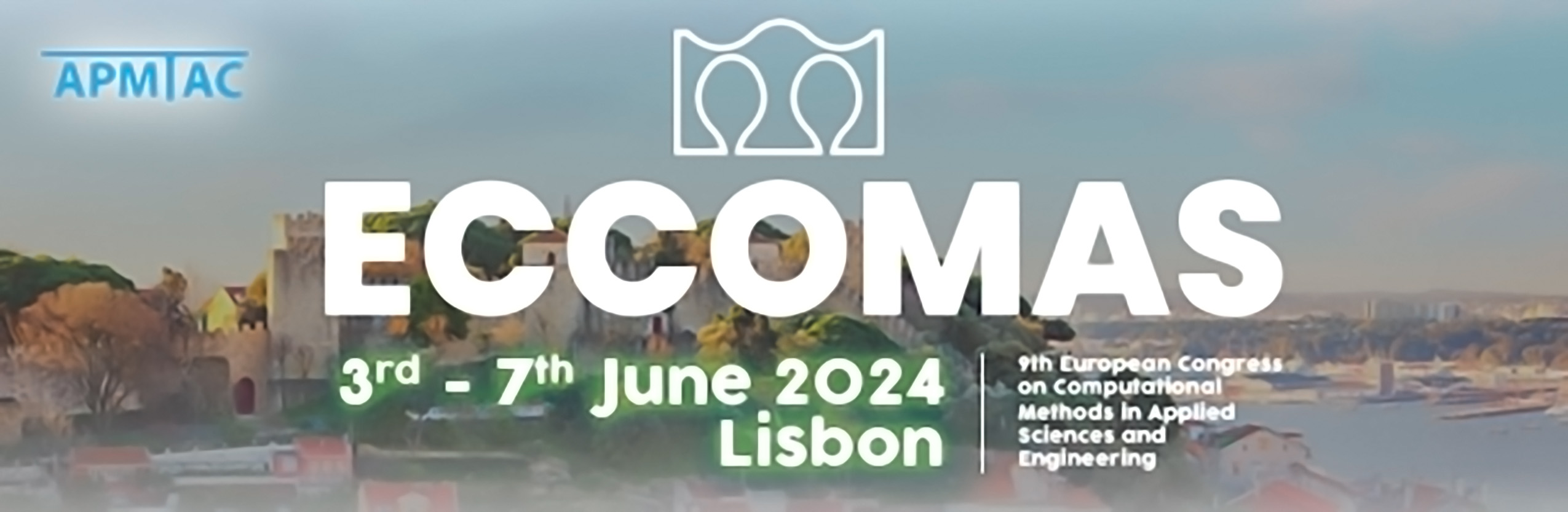
Machine learning-aided in silico model quantification of drug-induced viral mimicry response in cervical cancer
Please login to view abstract download link
In this work we propose and test a machine learning-aided agent-based modelling procedure for the investigation of anti-cancer, chemo-sensitizing and/or immunomodulating effects of decitabine on cervical cancer. The agent-based model (ABM) has been founded using BioDynamo, an open-source C++ platform designed for the development ABM simulations of biological systems. To ‘fit’ the model parameters on real data, we employ the Bayesian optimization algorithm, a method that optimizes an unknown, black box function. It consists of a statistical model that trains on the unknown function through observations and an acquisition function that indicates the exploration direction, i.e. which point to sample next. To obtain a ground truth based on real data, we rely on in-vitro cultivation of cells belonging to the HELA cell line. The duration of the trials spans 12 days in order to study cell viability in three distinct cases. The first is the case of the untreated cells and the second and third cases correspond to exposing cells to 0.3 μg and 1.0 μg of decitabine respectively. These measurements are used as the target for the exploration and optimization of the ABM parameters as well as for predicting the cell population dynamics to the decitabine and at different dosage options. To build our model we have experimented with the diameter of the cells as well as their potential for duplication and ageing to improve our model’s accuracy. We have also explored different distance functions for quantifying the correspondence of the various simulation results to the real-world data, in particular the Manhattan (L1 norm) and the Euclidean (L2 norm) distance. Our simulation results recapitulate the in vitro development of untreated cervical cancer cells, as well as cells exposed to 0.3 μg and 1.0 μg of decitabine respectively. We have accurately reproduced the cell viability time-graphs which illustrate the positive effects of decitabine at moderate to high doses. Furthermore, we perform a detailed analysis about our model’s efficiency with respect to the speed of the optimization, and the accuracy of ABM predictions using different distance metrics and for multiple cell dynamics’ model parameters.