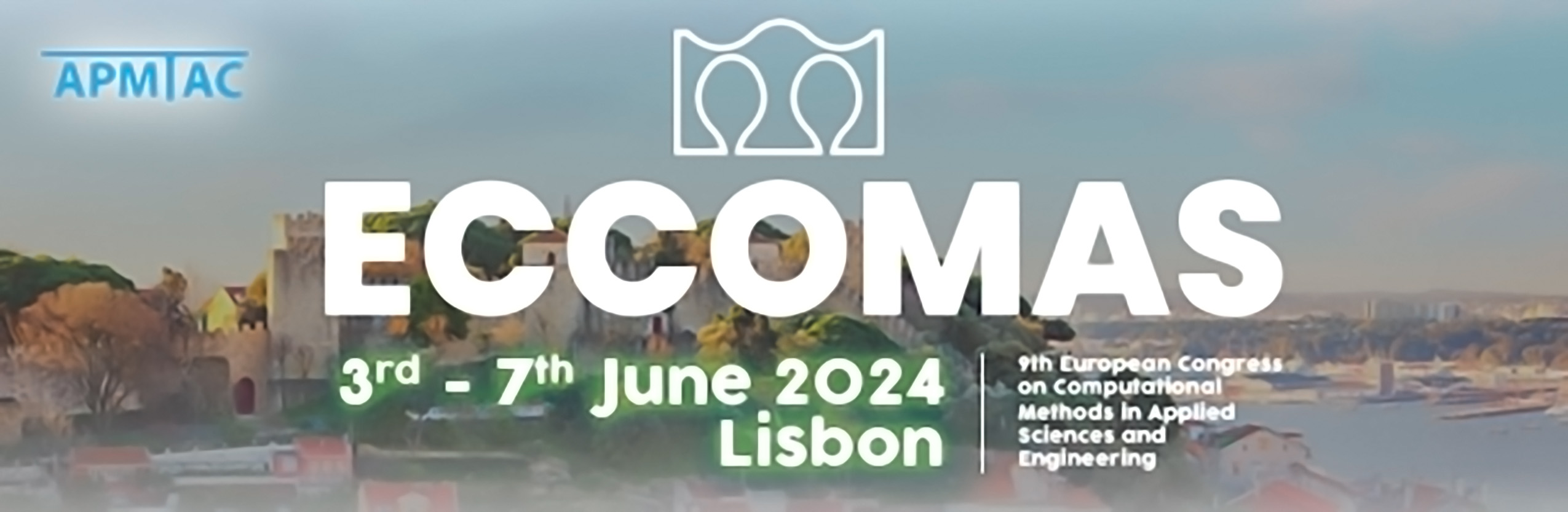
Belief Deep Markov Models for inference in complex partially observable decision-making problems
Please login to view abstract download link
Partially Observable Markov Decision Processes (POMDPs) offer the potential to model and solve complex sequential decision-making problems, including in the field of infrastructure management. Solution algorithms generally require - or highly benefit from - knowledge of the POMDP model, namely the underlying transition dynamics and observation generating processes. Additionally to training and testing the policy, in the context of partial observability, the knowledge of the POMDP model enables inference of a belief, namely a probability distribution over the system states. This drastically enhances the quality of the solution achieved. However, such a POMDP model may often not be available and its inference can be quite challenging. In this work, we propose a novel methodology, which importantly enables a model-agnostic POMDP inference and naturally scales to complex, continuous scenarios, and allows fast belief inference and dynamic updating of model parameters based entirely on available data (observations). The methodology builds upon the Deep Markov Model (DMM) architecture, which learns a broad class of state space models with deep neural networks trained with variational inference. Here, we introduce a novel model, termed the belief-DMM, which extends the DMM for adoption within the POMDP framework via incorporating actions and focusing on belief inference. We assess the efficacy of the proposed scheme in inferring the POMDP model structure and the associated beliefs in benchmark problems comprising both discrete and continuous state and action variables.