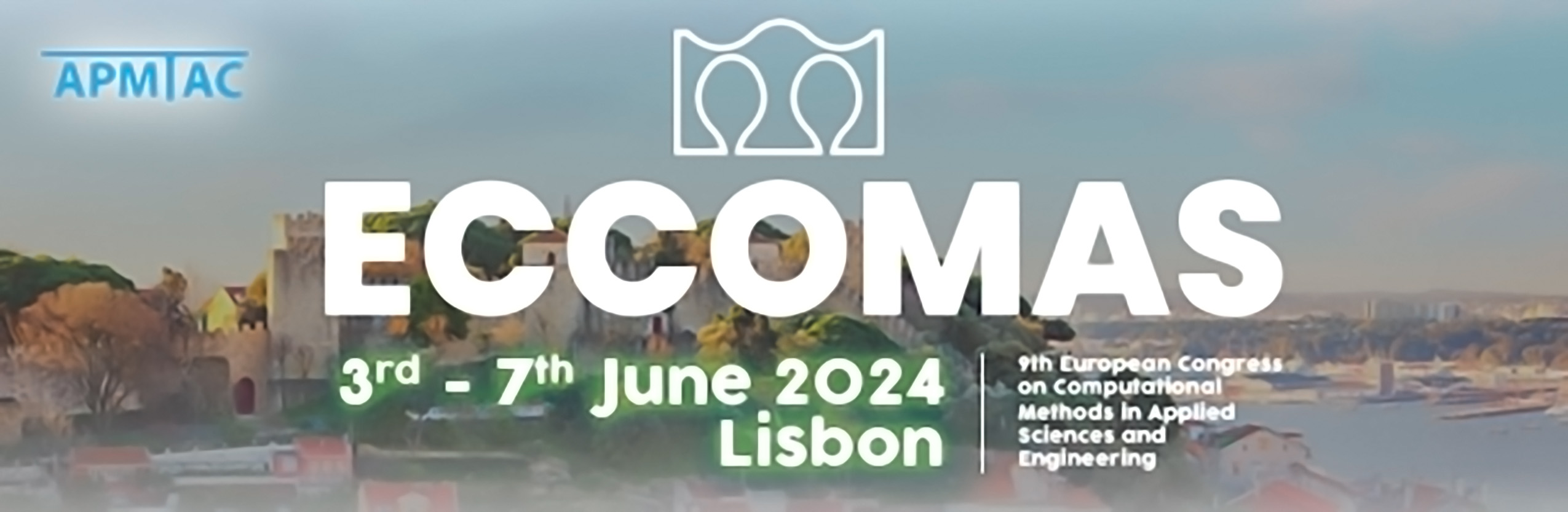
Why and how to incorporate physical information into artificial neural networks for mechanical problems
Please login to view abstract download link
Artificial neural networks (ANNs) and data-driven approaches recently have gained widespread popularity for solving computational problems, particularly also for the modeling of physical processes. In this context, ANN models emerge as fast, efficient and highly flexible alternatives to classic models. Recent advancements indicate that incorporating physical knowledge into ANNs can further enhance their performance. We achieve this by adapting the Rao-Blackwell theorem [1]. The theorem was established for statistical models and improves an initial estimator. It can be proven that the improved estimator yields a smaller or equal mean-squared-error compared to the initial estimator, making Rao-Blackwellization a powerful method that is guaranteed to never worsen an ANN. Our new framework enables the use of physical information, such as isotropy, observer invariance or dimensional analysis. It does so by using the initially statistical framework for a deterministic mechanical model. This new method moreover complements other approaches such as physics-informed neural networks (PINNs) [2], still preserving the optimality condition. Various aspects and advantages of the proposed method are demonstrated in terms of ANN design, data generation and data processing through a series of illustrative examples. The first example is a simple flow-curve prediction using an ANN, which serves to introduce the method. The subsequent examples are more complex and include the comparison of different improvement strategies for ANNs predicting elastic and brittle energy proportions based on a variational formulation to best use available data. A dimensional analysis of drilled steel bars is conducted to demonstrate how sufficient information can be obtained for characteristic forces. Finally, an ANN material model for rubber elasticity is constructed by incorporating isotropy and observer invariance. REFERENCES [1] G. Geuken, J. Mosler and P. Kurzeja, Incorporating sufficient physical information into artificial neural networks: a guaranteed improvement via physics-based Rao- Blackwellization. 2023. https://doi.org/10.48550/arXiv.2311.06147 [2] M. Raissi, P. Perdikaris and G. E. Karniadakis, Physics-informed neural net- works: A deep learning framework for solving forward and inverse problems involving nonlinear partial differential equations. J. Comput. Phys. 378, 2019. https://doi.org/10.1016/j.jcp.2018.10.045