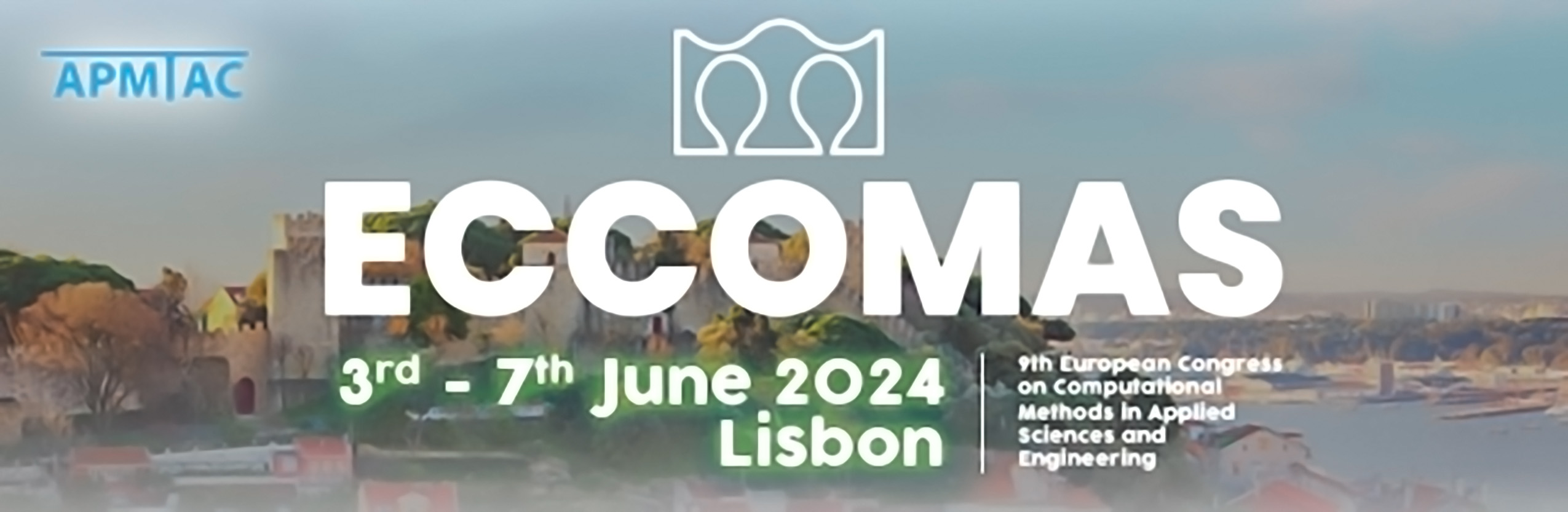
An integrated multi-imaging AI-based pipeline to study cardiovascular hemodynamic indices: aortic and pulmonary vessels case studies
Please login to view abstract download link
Statistical Shape Models (SSMs) are well-established tools for assessing the variability of vascular geometries. Several studies have been proposed, mainly focused on the aorta without supra-aortic branches . To overcome this limitation, also reflected in the subsequent Computational Fluid Dynamic (CFD) clustering studies, we developed a non-rigid registration algorithm to enhance the versatility of the SSMs. The potentiality of the code was also tested on a different vessel, the pulmonary artery. A total of 40 automatically segmented pulmonary arteries and 50 aortas were considered, originated from MRI and CT images respectively and extracted through a 3D UNet-based neural network. We developed an in-house algorithm for the non-rigid registration with: (i) a modified second order gradient descent approach, (ii) a loss function based on the minimization of Chamfer distance and (iii) four steps of remeshing. For the dimensionality reduction to few meaningful geometric features, we applied the principal component analysis. The first principal components were used to reconstruct 10 new geometries and to assess the effects of morphological variability on the correspondent CFD models. Simulations were carried out using ANSYS Fluent, considering the fluid as Newtonian and a laminar flow. For the hemodynamic analysis the wall shear stress, its related indexes (i.e., OSI, TAWSS) and the right/left flow split (only for the pulmonary arteries) were evaluated. A correlation was performed between the morphological and the hemodynamic features to derive a relationship between shape, fluid dynamics and pathology. The automatic segmentation reaches a mean Dice score of 0.93 for the CT and of 0.85 for the MR and allows to extract patient-specific information almost real-time (~30 sec). Although most of the target geometries presented very peculiar shapes, a maximum error of 0.5 and 1 mm in the registrations was observed in the aorta and pulmonary artery, respectively.