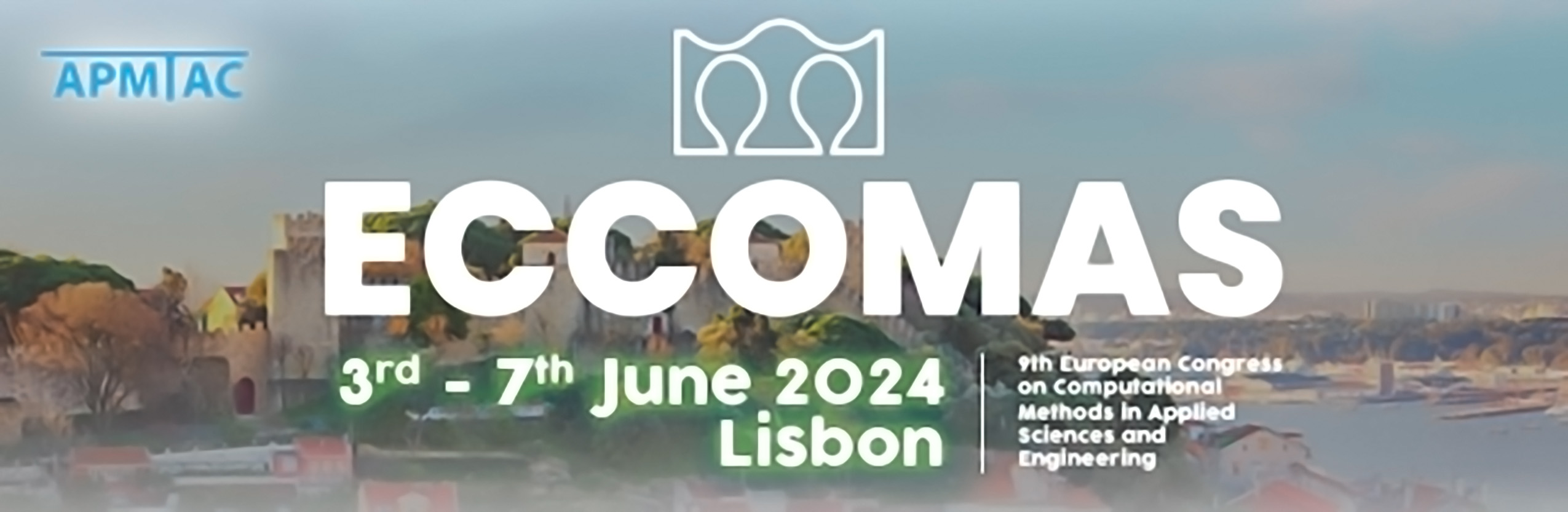
Designing unit cells with specific elastic properties with neural network
Please login to view abstract download link
One of the key strengths of neural networks lies in their ability to perform complex non-linear mappings between input and output data. This work focuses on training a neural network to determine the optimal unit cell topology corresponding to a specific constitutive elastic matrix. The neural network, designed to reverse the homogenization process, establishes a link between the unit cell's shape and its elastic properties. Utilizing a diverse range of geometries, a dataset was developed, that correlates elastic properties with their respective geometries. This dataset was then used to construct and train a feed-forward neural network using the deep learning toolbox available in MATLAB. The study involved homogenizing all geometries under periodic boundary conditions. The lattice was modeled as a biphasic material where the solid phase inherited the material properties, and the rest of the representative volume element (RVE) was treated as a void phase, assigned 1e-06 of the solid material's Young's modulus. For the effective imposition of periodic boundary conditions, a uniform mesh of 2D quadrilateral elements was employed. The neural network demonstrates its prowess by proposing suitable unit cells. Its ability to non-linearly map the relationship between a unit cell's elastic properties and its geometry significantly reduces the computational burden associated with conducting structural optimizations. This process effectively produces unit cells that possess the desired properties.