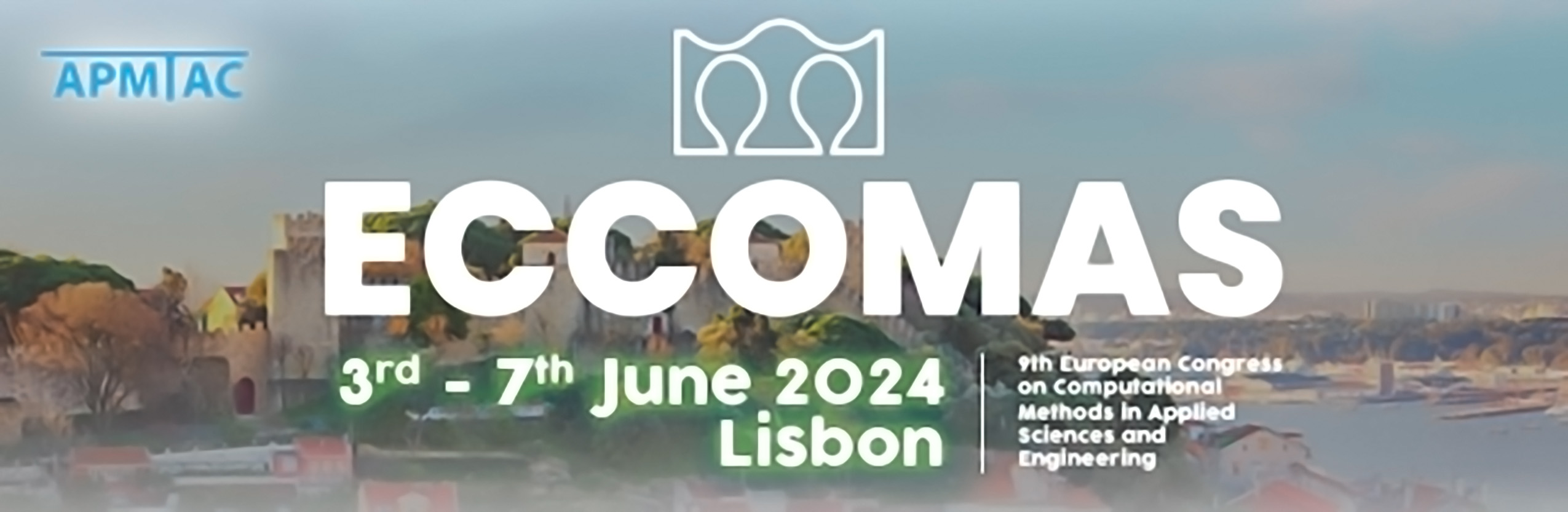
A Sequential Data-Integration Approach for Uncertainty Reduction in Hydro-Poromechanical Geoscience Models
Please login to view abstract download link
The awareness about the critical importance of dealing with uncertainty in numerical models is spreading over an increasing number of application fields, including geomechanics for energy resources. Sources of uncertainty are related, for instance, to the mathematical law governing the rock behavior, the geomechanical parameters and the geological nature of the field. By combining hydro-mechanical models with successive steps of data assimilation processes, we define an integrated model for the management of active reservoirs, used for both production and storage purposes [1]. The essential elements are: (i) a robust high-fidelity hydro-poromechanical Finite Element model of the field, (ii) a reliable surrogate model for the efficient construction of ensembles of realizations, and (iii) an ensemble-based data assimilation technique, such as the Ensemble Smoother. The proposed approach automatically integrates real measurements by successive data assimilation steps and progressively reduces the prediction uncertainties by a continuous training in time. In this communication, we present a set of real-world applications aimed at land subsidence prediction over off-shore hydrocarbon reservoirs in Italy [2]. In particular, we will consider sites where the primary production is still ongoing and where the post-production stage has to be managed. The analysis shows the robustness and reliability of the proposed approach, which builds a comprehensive geomechanical model of the investigated reservoir that may represent an essential tool to support decision-maker actions for the environmental and socio-economic safety. REFERENCES [1] L. Gazzola, M. Ferronato, M. Frigo, C. Janna, P. Teatini, C. Zoccarato, M. Antonelli, A. Corradi, M.C. Dacome, S. Mantica. A novel methodological approach for land subsidence prediction through data assimilation techniques. Computational Geosciences, 25(5):1731–1750, 2021. [2] L. Gazzola, M. Ferronato, P. Teatini, C. Zoccarato, A. Corradi, M.C. Dacome, S. Mantica. Reducing uncertainty on land subsidence modeling prediction by a sequential data-integration approach. Application to the Arlua off-shore reservoir in Italy. Geomechanics for the Energy and the Environment, 33:100434, 2023.