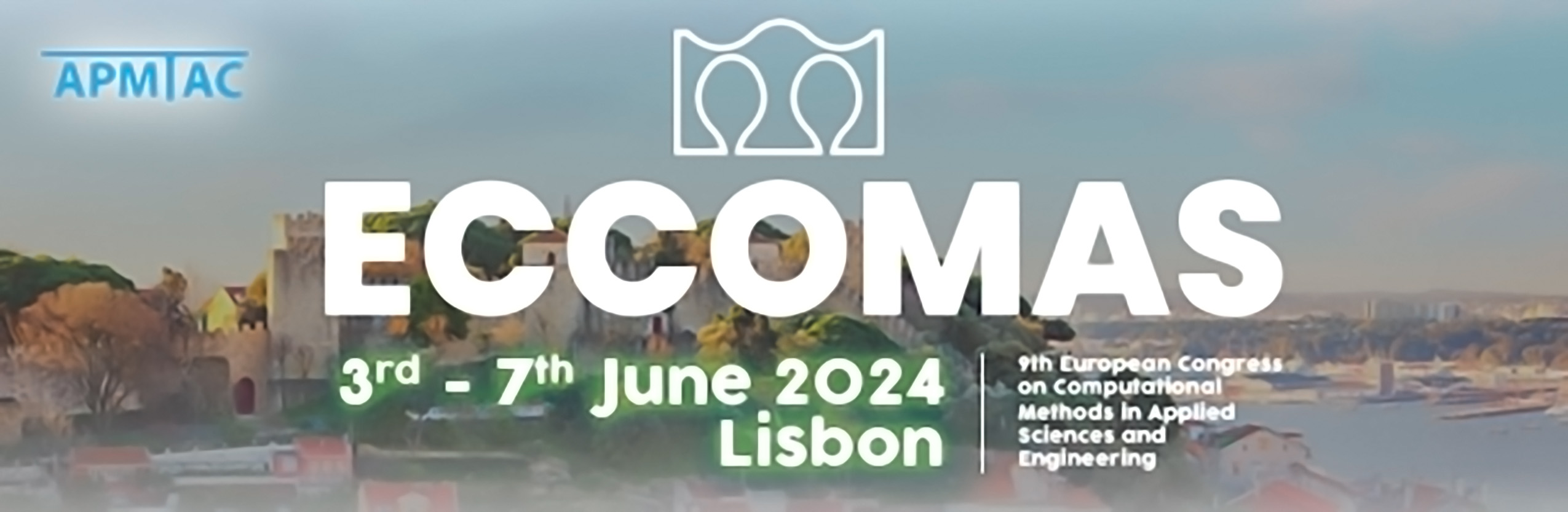
A data-driven framework to establish surrogate constitutive models of porous elastomers
Please login to view abstract download link
Porous elastomers exhibit highly nonlinear and path-dependent material responses to large deformations. Predicting such complex behaviours analytically is unfeasible; therefore, traditional approaches often rely on test data to model these materials. Recent advancements in homogenization theory have enabled the development of high-fidelity predictions for complex material systems. Researchers have leveraged various machine learning techniques to establish constitutive models based on data containing homogenized material responses obtained from high-fidelity micromechanical simulations [1-3]. In this research, we introduce a data-driven computational framework to implement surrogate constitutive models for porous elastomers undergoing large deformations. Explicit finite element (FE) simulations are conducted to compute the homogenised response of a cubic unit cell of an elastomer with constant initial porosity and material properties, subjected to a randomly imposed set of multiaxial strain states. The path-dependent stress response is predicted incrementally based on the current states of stress and strain, the prescribed strain increment, and the corresponding time increment. Average stress-strain measurements from FE simulations are utilized to assemble a training dataset for the surrogate macroscopic material model using simple neural networks. The prediction domain is gradually expanded to explore unseen initial porosities and material properties through active transfer learning. In addition to global stress predictions by the macroscopic surrogate model, the proposed framework approximates the corresponding local stress distribution in a porous unit cell via a coupled autoencoder architecture. The effectiveness of the proposed framework is demonstrated in producing material models with good accuracy and efficiency through several examples.