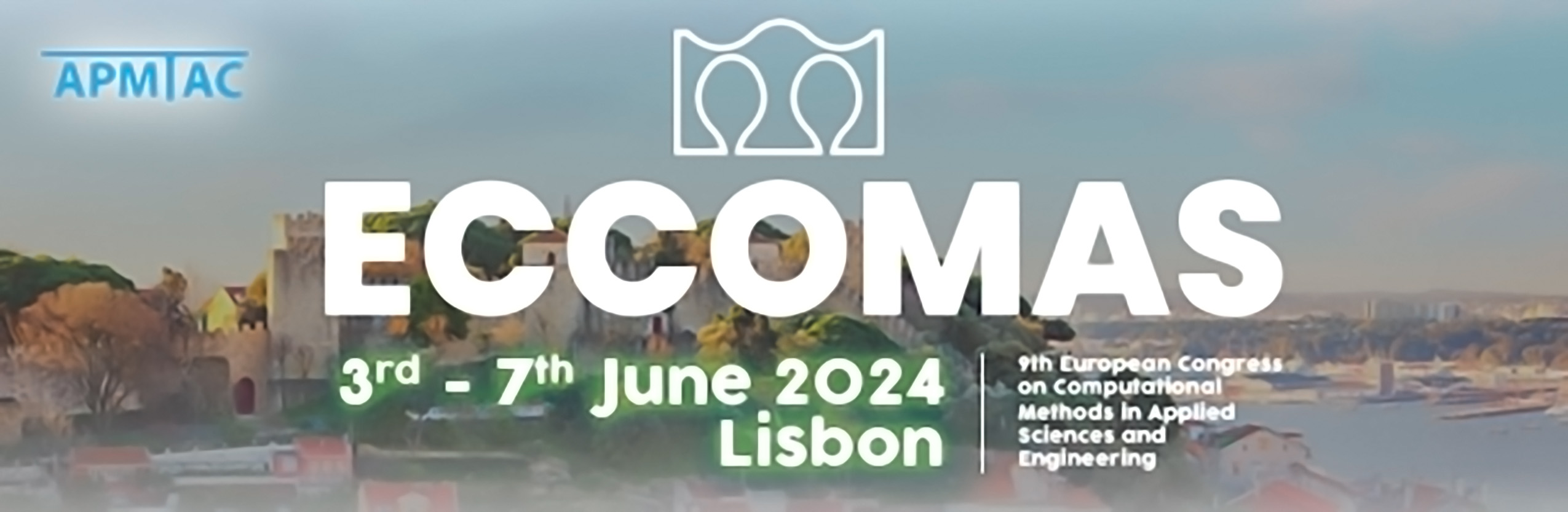
Data-Driven Structural Health Monitoring of Beam Elements Using Machine Learning Techniques
Please login to view abstract download link
Structural health monitoring in civil engineering applications has been rapidly growing and one of the current research challenges is to develop methods to identify damage for effective maintenance and retrofitting. This paper investigates the performance of different machine learning algorithms in damage identification of beam-like structures. Experimental studies were conducted on steel I-beams, introducing various damage scenarios by cutting the section at multiple locations and for different depths. The ambient vibration response was recorded using two sensing systems with different characteristics. Modal information was then extracted to calibrate numerical models, which were used to create a database of simulated acceleration records corresponding to several damage scenarios. For each, frequencies and modal shapes were extracted through stochastic subspace identification and frequency domain decomposition methods. The database was used to train and validate various machine learning algorithms, including multi-layered perceptron artificial neural network, support vector machine, and random forest. Based on the modal information, the outputs of the algorithms are estimates of location and damage severity. The study compares the efficiency of using each algorithm in localizing and quantifying damage, addressing classification and regression issues, and discussing the quality of data obtained from different monitoring sensors.