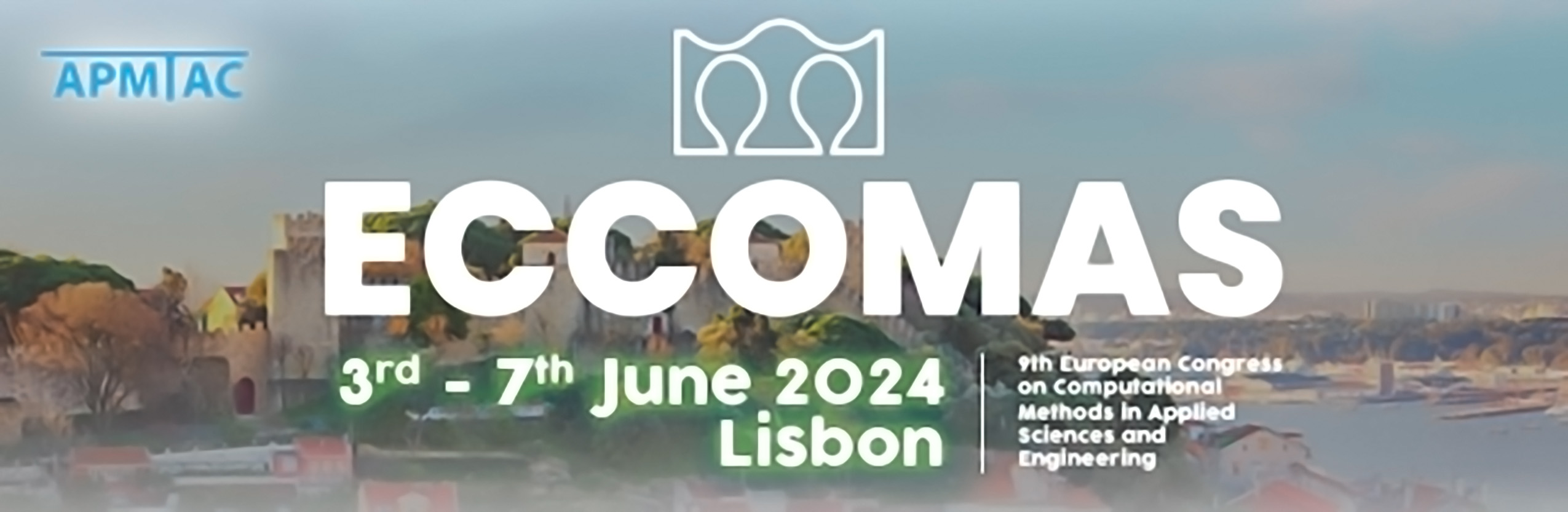
Simulation of ground penetrating radar data for railway condition assessment via Physics-Informed Neural Networks
Please login to view abstract download link
Traditional techniques to assess the degradation of railway tracks are intrusive, expensive, and time-demanding, hindering the implementation of proactive maintenance strategies. Recent research explores the use of Ground Penetrating Radar (GPR) as an efficient and non-destructive alternative to estimate ballast fouling and sub-surface moisture levels. While machine Learning and, in particular, deep learning models offer tremendous potential to automatically detect these features from GPR observations, these methods demand substantial amounts of labelled data. Moreover, generating extensive GPR datasets via simulation tools like gprMax is computationally intensive. To address these challenges, we introduce a new physics-informed neural network architecture that acts as a surrogate model for a physics-based simulator, namely gprMax, exploiting Maxwell's equations to efficiently simulate electromagnetic wave field propagation. Additionally to the novel characterization of the network, the proposed methodology potentially promises the fast generation of extensive, physics-based, labelled datasets. To this end, we evaluate the computational efficiency of our proposed model compared to the original simulation tool gprMax, which relies on use of a Finite-Difference Time-Domain method, thereby necessitating substantial computational cost. Our proposed solution, which relies in mixing of physics information with real world data, will be instrumental in enabling practical exploitation of advanced monitoring techniques for railway infrastructure maintenance.