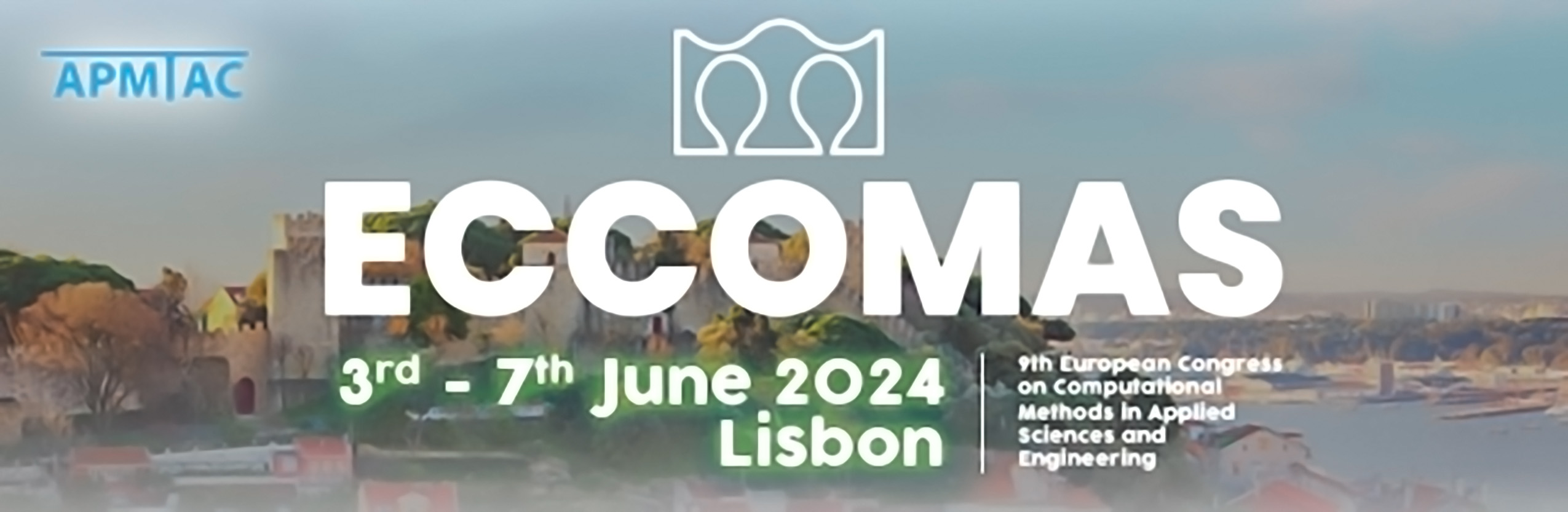
Machine-Learning-Based Surrogate Material Models For Elasto-Plasticity And Elasto-Damage
Please login to view abstract download link
Constitutive material models are used in the Finite Element Method (FEM) to determine the material response at each integration point. For complex materials, e.g., microstructured materials like composites, it is often necessary to fully resolve the microscale to reliably deduce the materials’ mechanical responses. One popular approach is FE^2 , where at each integration point of the macroscopic FEM model a microscopic FEM model is solved concurrently. This is however computationally extremely expensive, especially for three-dimensional structures with material non-linearities. The cost of solving the microscopic FEM models can be avoided by using a surrogate material model instead. For example, simple models can be used as surrogates for linear-elastic responses of the microscopic material. In contrast, if non-linear responses including dissipative mechanisms need to be considered, defining surrogate models is not as straightforward. Especially, if the material model should also be capable of predicting multi-directional loading paths with unloading. For such use cases, data-driven surrogate material models can be trained by utilizing training data produced from FEM-simulation results. In detail, for a body-centered cubic lattice material, microscale elasto-plastic simulations are conducted. The resulting machine learning (ML)-based surrogate of the lattice material is tested by comparing the corresponding macroscopic simulation results to fully-resolved simulation results. As a second use-case, the developed methodology is employed to convert an existing phenomenological analytical material model into an ML-based surrogate material model. This pre-existing material model has implemented elasto=damage mechanisms for unidirectional-reinforced composite materials, that are to be modeled in the surrogate material model as well.