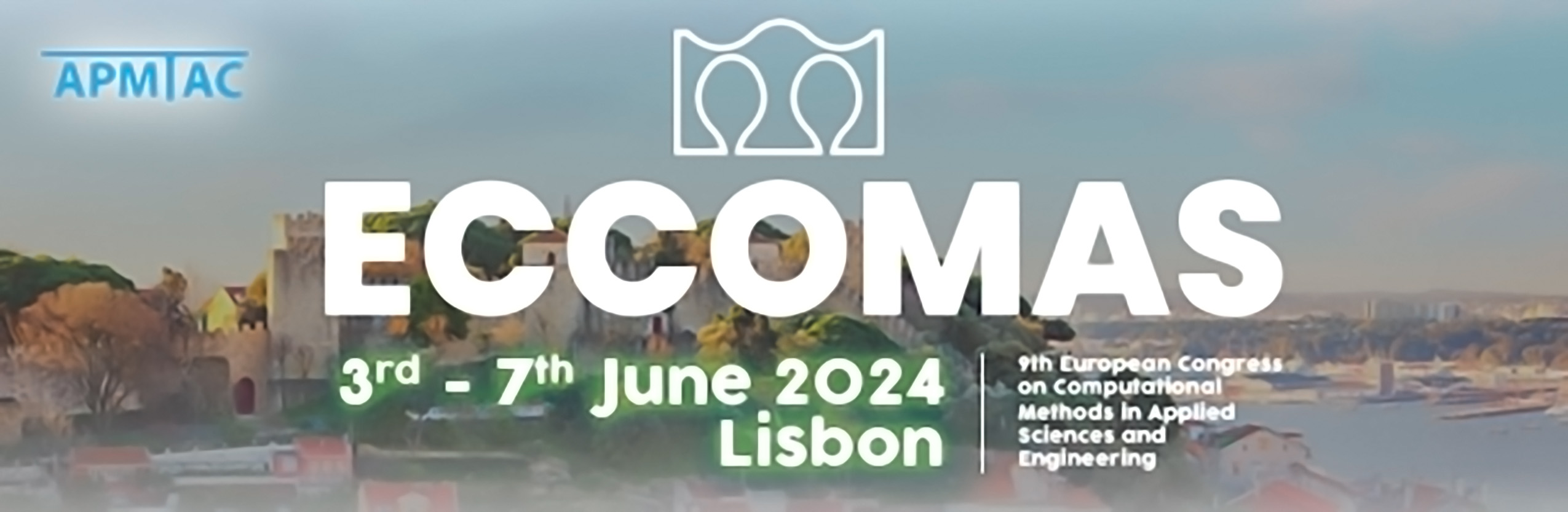
An Optical Data Innovative Format: Application to Land Cover Analysis
Please login to view abstract download link
Satellite data are foundational to Earth Observation (EO) programs that collect both optical and SAR (Synthetic Aperture Radar) images to monitor various natural phenomena, thus aiding in the management of Earth’s environment. Nevertheless, the rapidly increasing volume of EO data poses a challenge for efficient transmission to Earth. We present a novel compression method for multichannel optical data, that achieves very high compression ratios while guaranteeing a good representation accuracy. This goal is achieved by leveraging advanced mathematical tools. Specifically, the innovative approach includes a step of compressing information using Principal Component Analysis, followed by an anisotropic mesh adaptation procedure. This is done to accurately capture variations in pixel brightness across optical channels. Notice that the anisotropic adaptive representation of an image serves as an additional image compression step, achieving a notable reduction in the number of triangular tiles compared to the total number of pixels in the optical data. The transmission to Earth of such newly formatted satellite products entails customized encoding and decoding phases. The former stage is based on Hilbert curves and Huffman encoding; the latter resorts to Delaunay triangulations tailored to the anisotropic setting at hand. In this presentation, we provide an overview of the innovative compression procedure, by focusing on its building blocks. Moreover, we numerically evaluate the efficiency of the proposed optical data format in terms of i) compression and ii) information preservation capabilities. For the first task, we demonstrate the method’s remarkable performance, particularly when compared with the standard JPEG technique. Concerning ii), we assess the method in terms of classification accuracy by using learning models (Support Vector Machines and Convolutional Neural Networks). This task is performed both at the Earth level after decoding and, in a more computationally efficient strategy, on-board before the encoding phase. This performance evaluation is conducted using real satellite multispectral images, with the aim of addressing applications related to land cover.