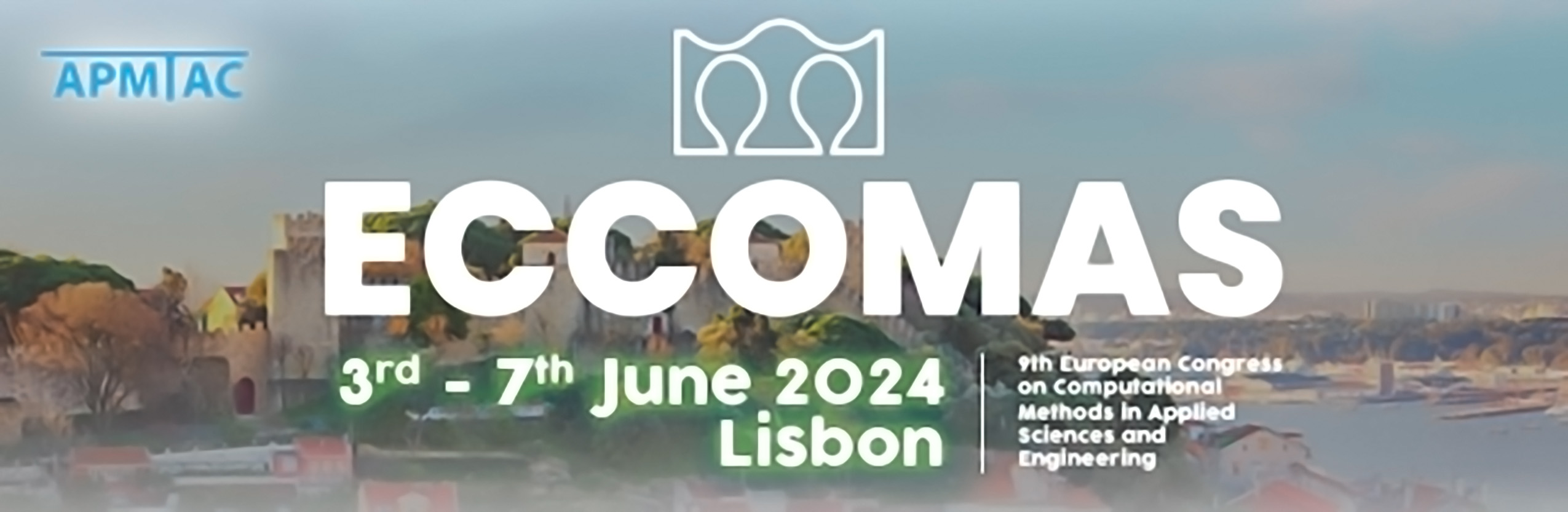
Super-Resolution of 4D-Flow MRI in the Left Ventricle Using Physics-Informed Neural Networks
Please login to view abstract download link
4D-flow magnetic resonance imaging (MRI) provides the capability to gather time-resolved, 3D reconstructions of haemodynamic flow for individuals in vivo. Current technology has several limitations however, including relatively low spatio-temporal resolution, noise artefacts and long scan times. Furthermore, clinically relevant quantities such as pressure, vorticity and wall shear stress (WSS) are not directly measured. In this work we present a novel super-resolution technique that builds upon recent developments in physics informed neural networks (PINNs) to produce recovered flow fields which are constrained by the Navier-Stokes’ equations and deliver sufficiently accurate velocity-pressure representations to allow derived quantities such as vorticity and WSS to be computed. The proposed technique is validated using synthetic flow data computed on CT-acquired patient-specific geometries of the left ventricle over a complete cardiac cycle. The CFD data is down-sampled in both space and time, with noise added, to simulate typical losses associated with 4D-flow MRI acquisition, and the recovered flow fields are shown to be of high quality. The impact of loss of resolution in the boundary geometry is also considered, with the proposed approach shown to be robust for key flow metrics (such as vorticity and kinetic energy) away from the smoothed boundary. Finally, computations are undertaken using clinical data to illustrate the proposed workflow to recover enhanced flow fields from raw 4D-flow MRI data.