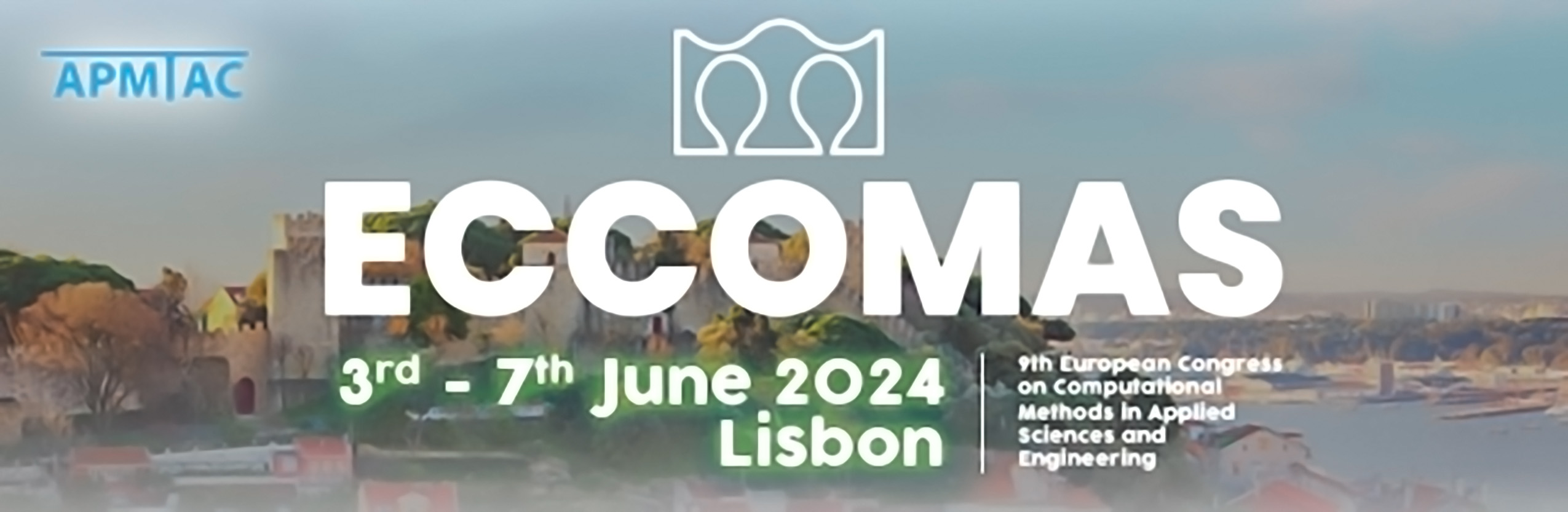
Structural Integrity Management at Scale through Deep Reinforcement Learning
Please login to view abstract download link
Deep reinforcement learning (DRL) allows us to manage our built environment beyond human cognition. We can now create digital twins that not only make sense of data and predict future structural responses, but also decide when, where, and how to intervene. Instating this autonomous decision-making process is, however, a complex computational problem. We need to concurrently (i) assess the value of noisy data to choose inspection and monitoring strategies; (ii) update our engineering models based on new knowledge and measurements; (iii) optimize state-altering interventions based on their causal effects on structural health and availability of resources; (iv) propagate uncertainties so that future risks are quantified and bounded; and (v) do the above for all components of a system considering spatiotemporal dependencies. In this talk, we discuss how DRL-driven structural integrity management, coupled with digital simulators, Bayesian inference engines, and partially observable Markov decision processes, can provide a rigorous basis for unifying the above tasks optimally, safely, and interpretably [1, 2, 3]. Emphasis is given to recent decentralized and hierarchical multiagent actor-critic architectures, which tackle large decision problems, otherwise intractable by other methods. We further reflect on the sensitivity of such autonomous systems to changes in information availability, multi-agent coordination, constraint stochasticity, and task hierarchies, to understand emergent risks and inform modeling practices. Applications in diverse systems, from transport assets to wind farms, among others, highlight the major socioeconomic benefits we can reap, and point to missing steps forward. REFERENCES [1] Andriotis, C.P., Papakonstantinou, K.G., 2019. Managing engineering systems with large state and action spaces through deep reinforcement learning. Reliab Eng & Sys Saf, 191. [2] Andriotis, C.P., Papakonstantinou, K.G., 2021. Deep reinforcement learning driven inspection and maintenance planning under incomplete information and constraints. Reliab Eng & Sys Saf, 212. [3] Morato, P.G., Andriotis, C.P., Papakonstantinou, K.G., Rigo, P., 2023. Inference and dynamic decision-making for deteriorating systems with probabilistic dependencies through Bayesian networks and deep reinforcement learning. Reliab Eng & Sys Saf, 235.