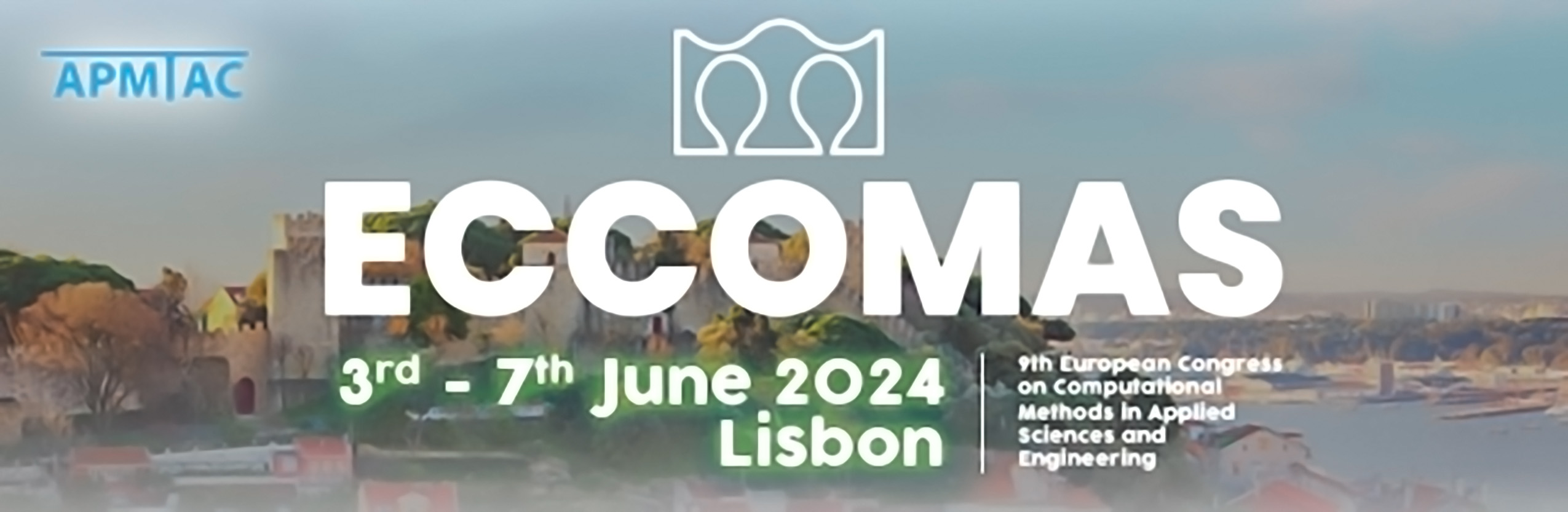
Machine learning and statistical shape modelling for real-time prediction of stent deployment in realistic anatomies
Please login to view abstract download link
One of the preferred endovascular options for treating intracranial aneurysms (IAs) involves deploying a very dense braided stent at the aneurysm neck to isolate it from the blood circulation. Treatment planning mainly relies on measurements from images acquired shortly before surgery. Being highly dependent on the practitioner’s experience, this process is prone to errors that can lead to potential complications during and after the operation. Computational models, particularly finite element (FE) simulations, are becoming powerful tools in clinical practice to examine different treatment options and anticipate potential causes of failure [1]. However, the complexity of the problem under exam makes computational time unsuitable within the clinical timeline of IAs treatment. To address these time constraints, we propose to integrate FE simulations with machine learning (ML) methods. The framework builds on previous work to develop an efficient FE solver in Julia for modelling contact interactions between wire-like structures, discretised using beams, and rigid surfaces, described using a signed distance field [2]. Thanks to an efficient Julia code and dedicated modelling strategies, the computational cost of braided stent deployment simulations was significantly reduced. This allowed the rapid collection of large simulation databases, crucial for ML training. The concept behind this work is that, if the geometry in which the stent is deployed is parametrised, a ML model can be trained to establish a mapping between these parameters and the corresponding stent configuration obtained by FE simulation. Once properly trained, the model should be able to provide accurate real-time solutions for unseen geometries. This framework has been tested before using an idealised parametric model of an aneurysmatic cerebral artery, yielding promising results [3]. In this study, we extended this previous work by incorporating more realistic geometries. These are computed by means of a statistical shape model derived from a clinical database, capturing significant intra-subject variability in patient-specific cases. When testing the trained ML model, the errors between predicted and FE results fell within the same order as the spatial resolution of the images used for IAs treatment. This once emphasised the importance of further exploring the use of machine learning techniques for the clinical translation of computational tools.