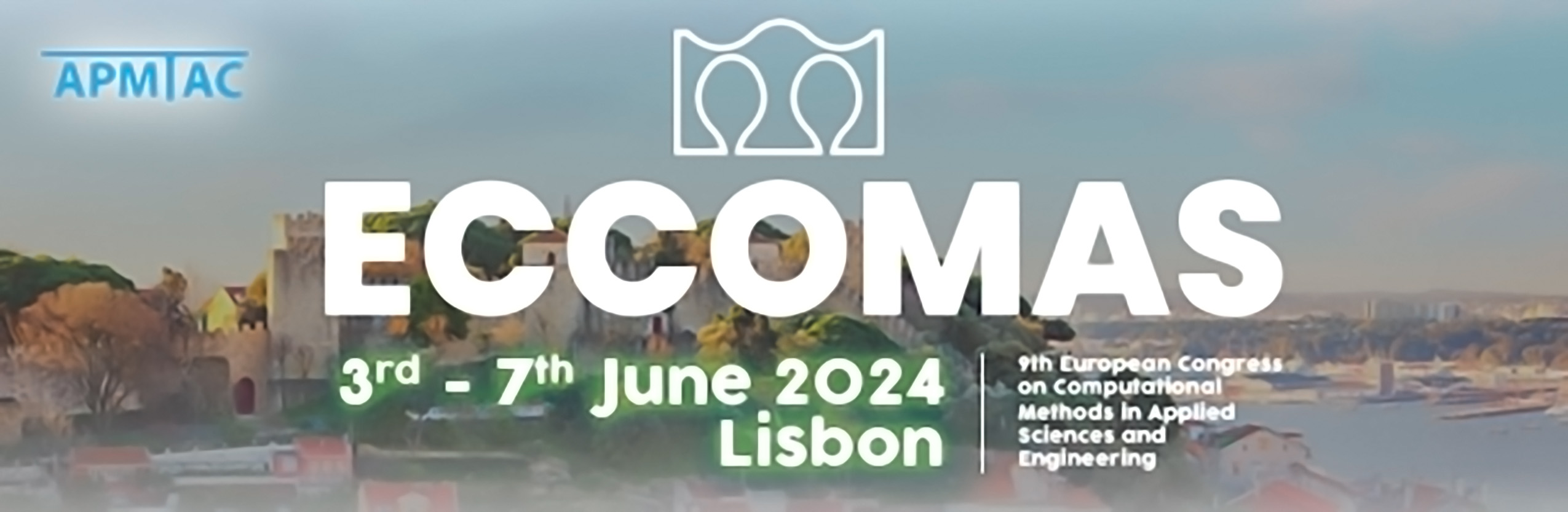
Approximating Fine-Scale Features in Time-Dependent Multiscale PDEs with Neural Networks
Please login to view abstract download link
Solving time-dependent partial differential equations in media that vary on a fine scale requires specifically designed multiscale methods to reduce the computational costs that arise for classical methods which need to resolve these fine scales. A numerical homogenization method that achieves this reduction is the localized orthogonal decomposition (LOD) method, where local sub-problems are solved in an offline phase to enrich coarse scale finite element basis functions to obtain good approximation properties without resolving the fine scale. This leads to rather small systems of equations needed to be solved which is especially useful when iterating through time. If multiple coefficients need to be analysed, solving the local sub-problems is the bottleneck of the method. We replace solving the local sub-problems with a physics-informed neural network (PINN) based strategy, which generalizes the methodology to multiple coefficients and still results in small systems of equations when solving the partial differential equation (PDE). We show that the PINN achieves good approximation of the enriched basis functions, and generalizes very well to a plethora of oscillating PDE coefficients. In this talk we present the basic idea of the method, and show some numerical examples of time-dependent PDEs.