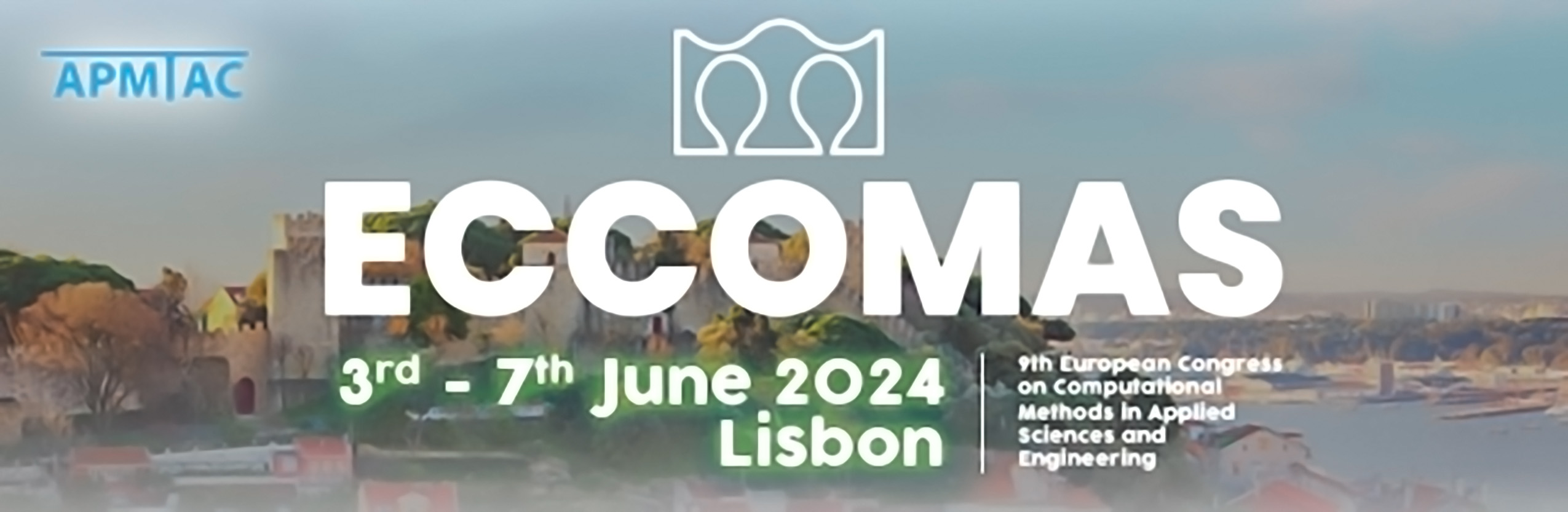
Machine Learning-Based Inverse Problem Solving for Identifying Heat Input in Tungsten Inert Gas (TIG) Welding
Please login to view abstract download link
Arc welding is a critical method for joining metallic materials by melting and solidifying them through the generation of an electric arc. However, this process can lead to defects such as cracking due to thermal stresses. To improve structural integrity and prevent defects, digital twin technology is valuable. It can create virtual models of welding processes . Structural integrity is primarily determined by the microstructure and residual stresses. Additionally, thermal dynamics play a crucial role in influencing stress states and inducing metallurgical changes in welded components. The thermal field essentially depends on heat input, which is influenced by welding process parameters. Predicting thermal fields based on these parameters can be achieved using two approaches: multi-physics modeling, which is detailed but computationally intensive, and equivalent heat source modeling. Although the latter doesn't capture the full complexity of the underlying phenomena, it still provides a comprehensive temperature field for most of the workpiece. Various equivalent heat source models exist, with efforts made to match experimental observations. Identifying unknown parameters can be achieved through inverse problems, where causes are determined from observed effects. This can be done deterministically using optimization algorithms or probabilistically to estimate parameter probability distributions. In our contribution, cameras are employed to monitor the welding pool shape during welding experiments using Tungsten Inert Gas (TIG) welding. The data generated through finite element analysis is used to create a surrogate model using machine learning regression, linking unknown parameters to weld pool characteristics. The trained surrogate model predictor is then used to formulate a cost function that describes how closely experimentally observed quantities of interest match those resulting from the modelization. Through the optimization algorithm, the cost function is minimized, providing a solution to the inverse problem.