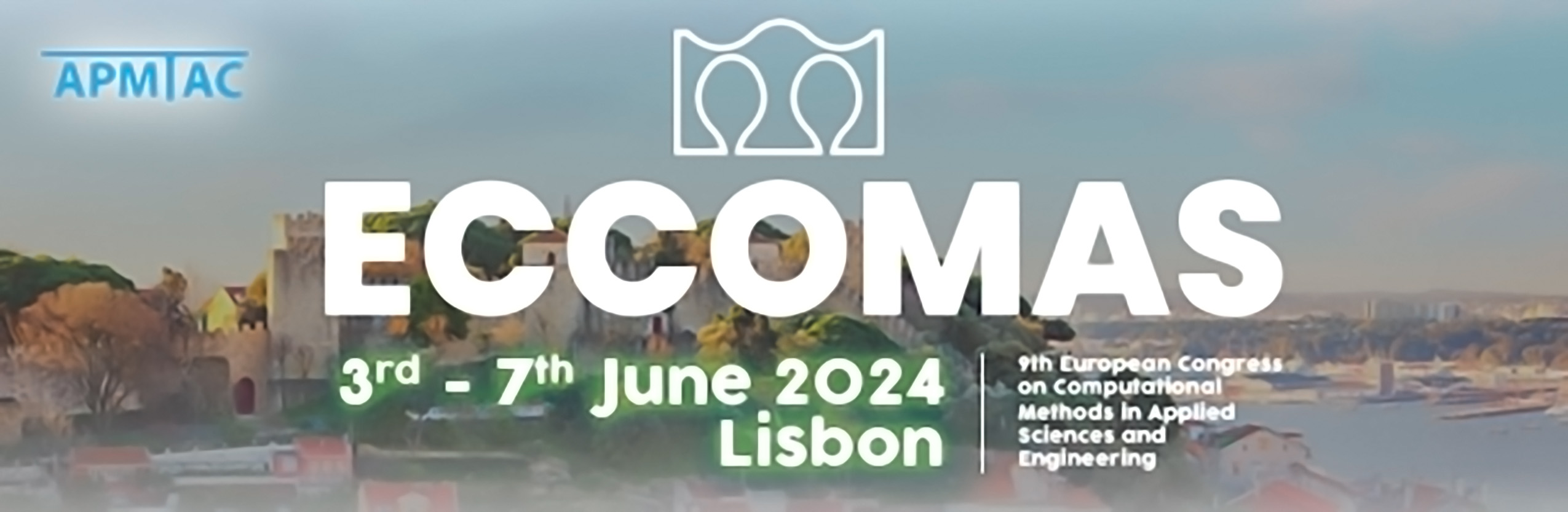
From Theory to Practice: Harnessing PIML for Enhanced PHM Capabilities
Please login to view abstract download link
In the rapidly evolving field of Prognostics and Health Management (PHM), integrating machine learning with fundamental physics principles, known as Physics-Informed Machine Learning (PIML), is emerging as a transformative approach. This keynote presentation delves into the intricacies of PIML, exploring its conceptual framework, methodologies, and innovative applications in PHM. Drawing insights from four pivotal studies, this presentation will unravel the terminologies and concepts central to PIML, providing clarity on its distinction from traditional machine learning and physics-based methods. A comprehensive taxonomy of PIML approaches used in PHM will be discussed, illuminating how physics-informed strategies enhance diagnostic and prognostic capabilities in diverse applications. Furthermore, innovative methods developed in our research will be showcased. They illustrate how novel PIML approaches can be effectively applied in PHM scenarios, thereby demonstrating the practical impact and potential of this field. Following the showcase of innovative methods developed in our research, the discussion will navigate challenges and opportunities that PIML presents in the PHM domain. This segment aims to shed light on the future directions of PIML research, particularly in addressing complexities such as sparse data, interpretability issues, and the integration of heterogeneous knowledge sources, which are pivotal in advancing the field and harnessing its full potential in PHM. In summary, the presentation aims to provide a comprehensive understanding of PIML, its current state, challenges, and future potential in PHM. By integrating both qualitative and quantitative insights, the talk will contribute to a holistic comprehension of PIML in PHM and its practical applications, offering attendees a roadmap for future research and development in this exciting and promising area of study.