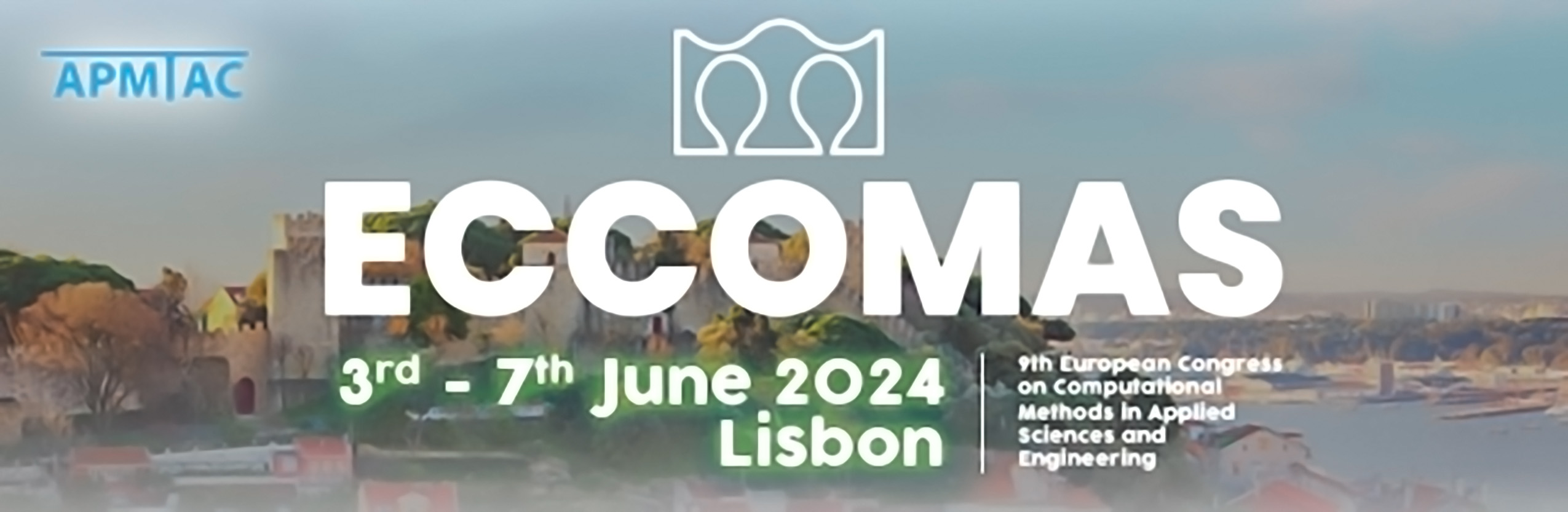
Reliability of a Load-Path Dependent Structure using Autoregressive Models
Please login to view abstract download link
Computing failure probabilities in time-dependent reliability analysis is a demanding task, particularly when rare-events are of interest, as in structural reliability. Structural loads are usually modeled as stochastic processes, whereas structural degradation can be modeled either as deterministic or stochastic. Many problems of this type can be addressed via well established techniques (e.g. fast probability integration, directional simulation, out-crossing approach, time-integrated approach [1]), which albeit efficient, are approximate or problem-type specific. Such solutions do not work in the context of load-path dependent reliability, where failure at a given time tau depends on the whole time-trajectory of structural loads up to time tau. This problem can be addressed via brute Monte Carlo Simulation, sampling all time-dependent loads with the help of series expansions methods, like Karhunen-Loève expansions (KL) and the expansion optimal linear estimation method (EOLE) [2]. This approach tends to be quite inefficient, and surrogate models have been employed to mitigate the high computational costs [3]. Although less common in this context, time series models [4] have been utilized in structural reliability for addressing specific problems. Recent studies have shown autoregressive models (AR) and autoregressive moving average models (ARMA) to achieve good precision with lower computational costs in the task of sampling stochastic loads [5,6]. In this work, autoregressive models are used to represent structural loads as time-series in a load-path reliability problem. Performance is evaluated and compared with solution via EOLE. Results suggest that AR models may be a good alternative for load-path dependent problems in terms of accuracy and computational costs.