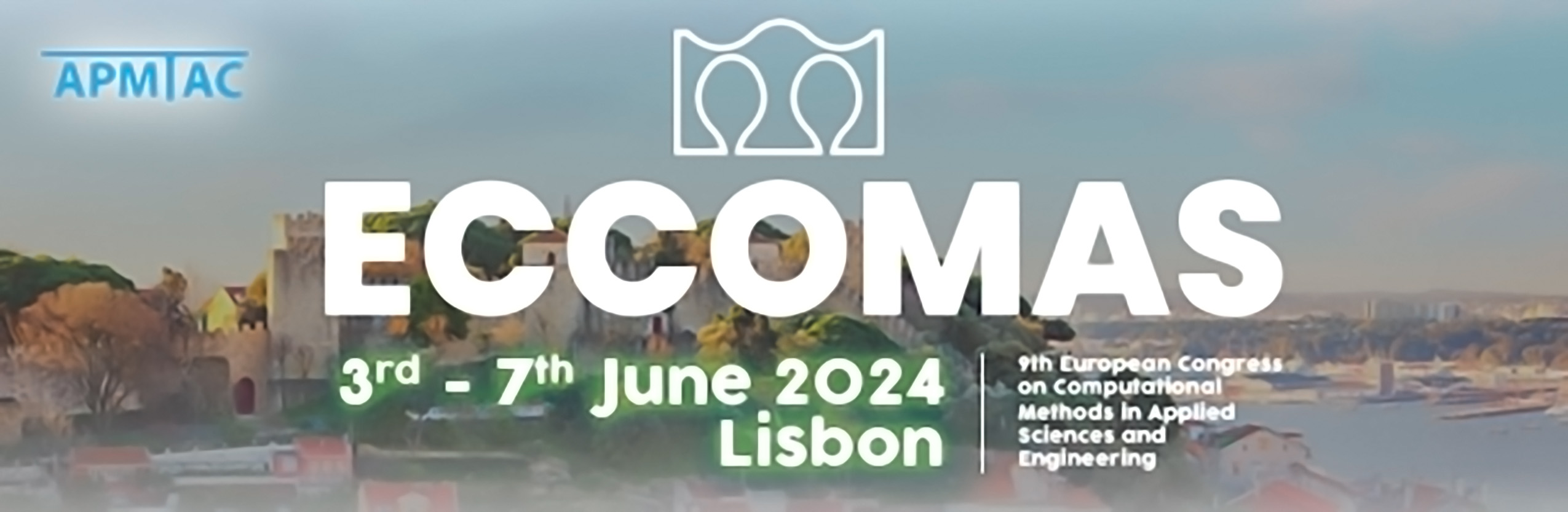
Improving weak PINNs for Hyperbolic Conservation Laws: Dual Norm Computation, Boundary Conditions and Systems
Please login to view abstract download link
Physics-Informed Neural Networks (PINNs) are a new machine learning method for approximating solutions to partial differential equations. They are based on minimizing a Monte-Carlo approximation of the L 2 norm of the PDE residual. However, for systems of nonlinear hyperbolic conservation laws, we show that standard PINNs fail at approximating discontinuous solutions using explicit computations. Our computations imply that it is necessary to use weak (dual) norms of the PDE residual instead, in analogy to moving from the strong form of the PDE to the weak formulation for discontinuous solutions. This approach has been termed “weak PINNs” (wPINNs), wherein an adversarial neural network estimates the dual norm. Extending wPINNs, we approximate the weak norm computation by solving a family of dual elliptic problems for the PDE residual. This makes learning more stable and effective. Similarly, we introduce and compute an entropy residual. Our modified wPINNs also extend naturally to systems of conservation laws. For the case of scalar nonlinear hyperbolic conservation laws we also outline how to treat boundary conditions in a weak sense using similar techniques and show the efficacy of this strategy. We verify our technique and extensions in numerical experiments for Burgers equation and the compressible Euler equations.