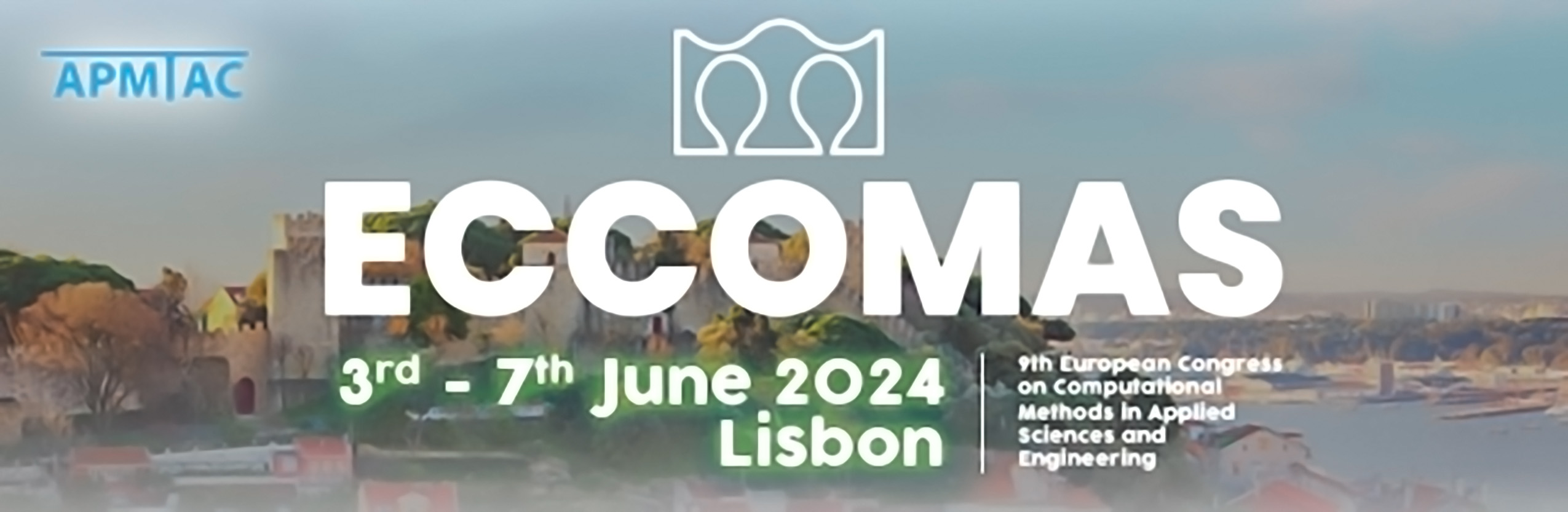
Human Exhaled Breath Physics Based User Identification
Please login to view abstract download link
Air flowing out of the lung interacts with the complex internal structures of the extrathoracic airway. In addition, the Reynolds number associated with this flow is sufficiently high, together leading to the complexity in the flow. We hypothesize that the structure of turbulence in the exhaled air is unique and identifiable from person-to-person, making the exhaled breath a biomarker. This study aims to build a biometric system that works purely based on the fluid mechanics governing exhaled breath. Methods including classical multi-dimensional hypothesis testing approach and machine learning models are employed in building user authentication algorithms, namely user confirmation and user identification. A user confirmation algorithm tries to verify whether a user is the person they claim to be. A user identification algorithm tries to identify a user's identity without prior information. A dataset of exhaled breath time series samples measured using hot wire anemometer from 94 human subjects was used to evaluate the performance of these algorithms. A class binarization approach was employed while building the algorithms, where classifiers were built for every pair of users in the dataset. The accuracy in binary classification of a majority of user pairs was above 60%, which signifies the existence of some uniqueness in an individual’s exhaled breath. The user confirmation algorithms achieved true confirmation rates of over 97% for the given dataset. The user identification algorithm performs reasonably well, with over 50% of the users identified as being within two possible suspects. Such a system can also be used as a tool to gain insights into the morphometric variation of extrathoracic airway across individuals. Such tools are expected to have future potential in the area of personalised medicines.