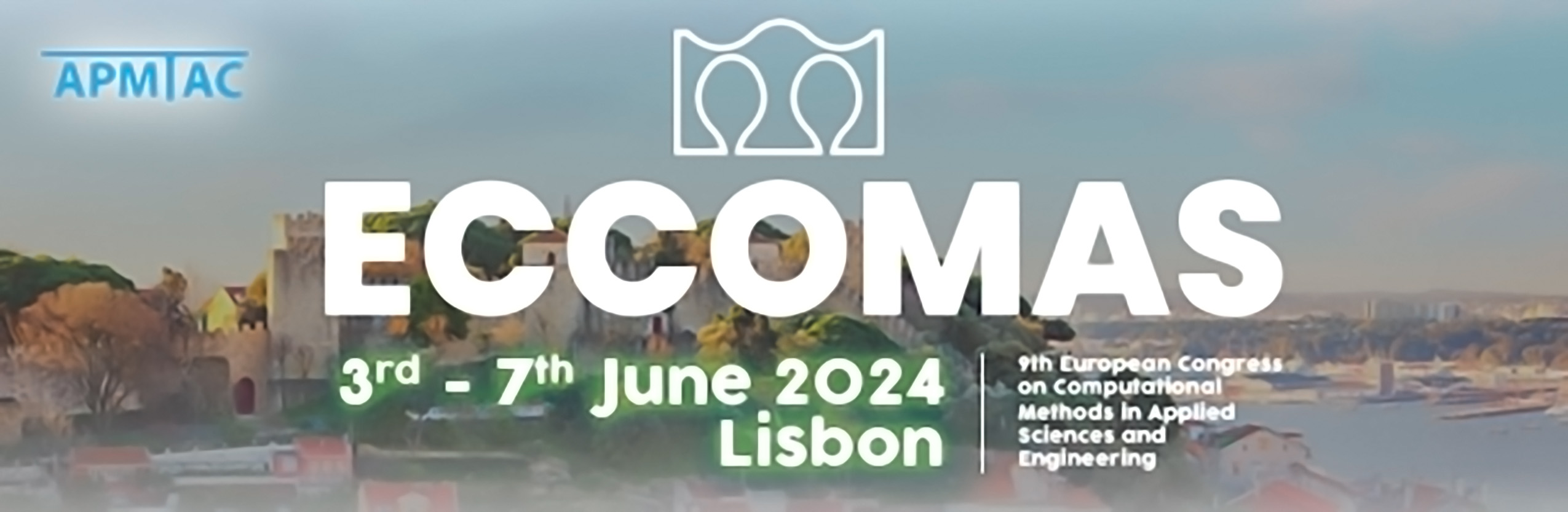
Adjoint-Optimized Deep Learning Sub-Grid Scale Models for Airfoil Flows
Please login to view abstract download link
There is a substantial interest in developing deep-learning sub-grid scale (DL-SGS) models to improve the predictive ability of large eddy simulation (LES) at coarse mesh resolutions. Most existing literature train DL-SGS models using standard regression, where the input to the neural network is the filtered/time-averaged DNS, the target data is the DNS’ SGS tensor, and training is performed off-line from the LES simulation. Once trained, the closure model is substituted into the LES for an a posteriori simulation. However, since the model was only trained on a priori DNS data, the training and prediction (LES simulation) are potentially inconsistent. (For instance, the model receives DNS data as its input during training while it receives LES data as an input during prediction.) In our approach, we use adjoint optimization to directly train over the entire DL-LES simulation. Specifically, we optimize the DL-SGS model to minimize the difference between the short-time evolution of the LES solution and the high-fidelity DNS data. This approach has been successfully implemented for incompressible flows for several canonical configurations. In this new research, we develop adjoint optimization methods to train DL-SGS models for compressible flows. A DL-SGS model is trained on a compressible flow around an airfoil at Reynolds number 50,000 with a 5 degrees angle-of-attack (AoA). Turbulent airfoil flows are substantially more complex than the previous canonical flows (e.g., isotropic turbulence and turbulent jets) for which adjoint-trained DL-SGS models have been evaluated. The adjoint-trained DL-SGS models are accurate when extrapolating to out-of-sample AoAs for airfoils. The model reduces errors in lift and drag coefficients by over 50% compared to classical SGS models when it has been trained over broadly representative flow physics, and its far out-of-sample results are competitive with the best classical SGS model tested. At the conference, we will also present models trained on multiple flow conditions and evaluations of the DL-SGS model’s out-of-sample accuracy for new geometries.