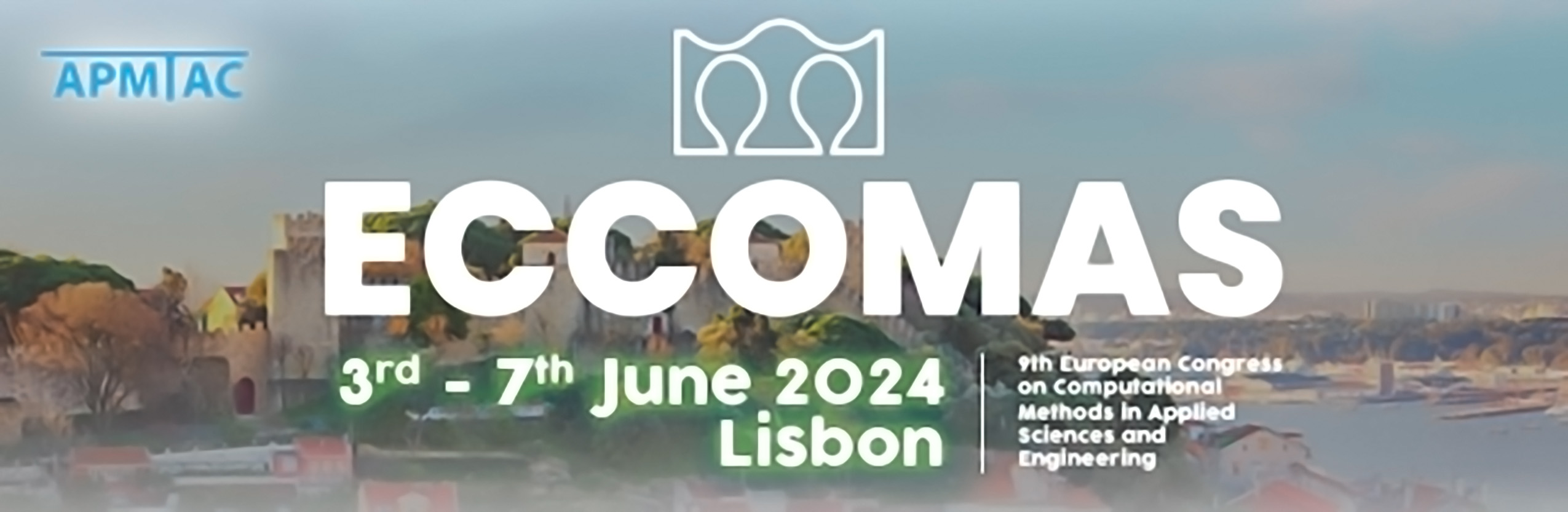
Bayesian Quality-Diversity optimization for conditional search-space problems based on budget allocation
Please login to view abstract download link
During the preliminary design and optimization of engineering systems, architectural and technological choices need to be made. In the mathematical formulation of the optimization problem, they are represented by design variables (called conditional variables) acting on the structure of the problem (e.g., number of design variables and constraints). Solving this Conditional Search-Space Problem (CSSP) requires to handle mixed-variables combinatorial design spaces. A broad diversity of solutions is available, but can not be captured by classical optimization algorithms that search for the best design (or tradeoffs between multiple objectives). Quality-Diversity (QD) is a novel branch of optimization providing a set of diverse high-performance solutions with respect to feature functions describing their characteristics, but is not adapted to deal with CSSP. This work proposes a strategy to solve CSSP by using a Bayesian QD approach. This consists in decomposing the problem in several subproblems with a non-conditional structure, on which surrogate models are built. A Bayesian optimization strategy coupled with a QD algorithm is used to search for potential solutions among the subproblems. Then, a budget allocation policy is defined to evaluate those potential solutions with the exact functions. The proposed methodology is evaluated on several analytical problems and a launch vehicle design problem. In this paper, the mathematical formulation of the CSSP in the QD context is described. The proposed solving methodology is detailed, and numerical simulations are performed to assess its performances. A comparison with a full exploration of all the subproblems with an equal budget distribution is given and a discussion is proposed to conclude.