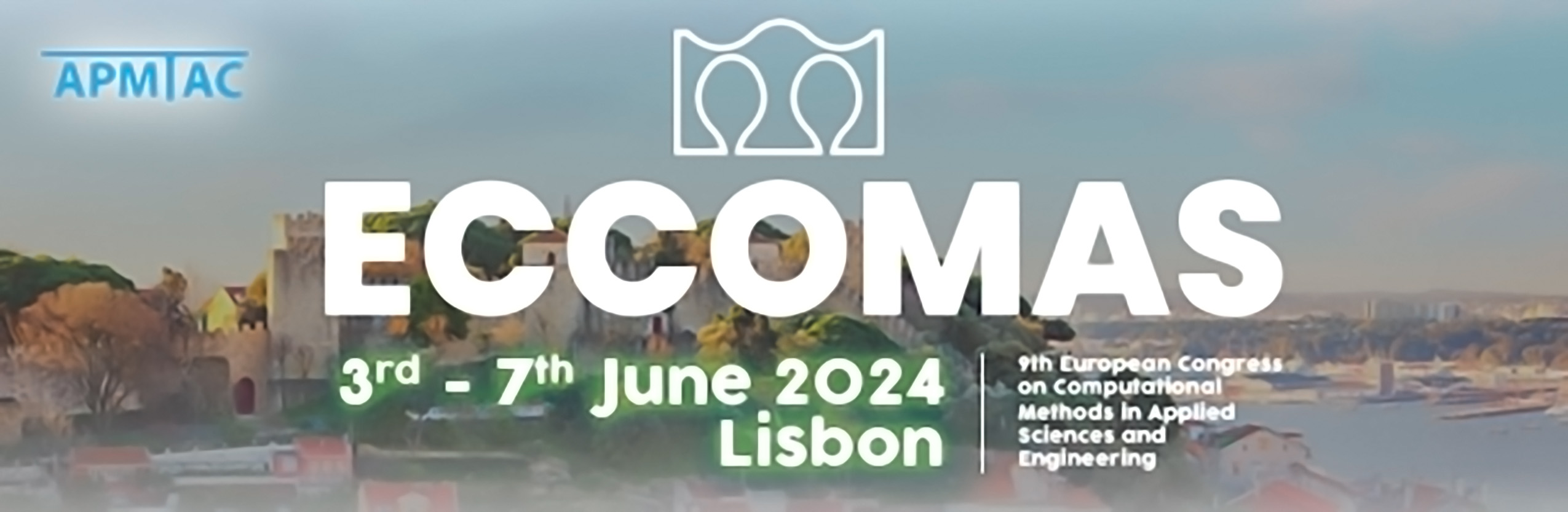
Walking parameter inference from strain data for a footbridge
Please login to view abstract download link
Accurate identification of structural loads is critical in digital twin applications such as structural health monitoring. Structures are rarely equipped with sensors that can adequately characterize the loads but may have sensors that measure the resulting response. In this work, we consider an indoor footbridge monitored by six strain gauges. We demonstrate that we can infer from strain data a detailed description of a pedestrian’s motion, including speed, stepping rate and walking path. We observe that the walking path has a significant impact on structural response. Furthermore, we observe that a higher fidelity walking path model (e.g., allowing for curved walking paths) improves our ability to infer other parameters of the pedestrian motion. The increased fidelity in the walking model incurs an increased computational cost to our parameter inference procedure, motivating the following contributions. Our first contribution is the development of a projection-based reduced order model (pROM) for the bridge which ensures rapid evaluation of the strain and its gradient with respect to the walking model parameters. In standard pROM training, we collect snapshots of the system response for representative load conditions and then construct the reduced model using the snapshots. However, the standard sampling procedure is intractable in our case because an attempt to sample the space of all possible bridge traversals results in too large of a training set. Instead, we exploit that the walking load is decomposable into individual footfall loads, and develop a pROM training procedure that takes advantage of such a decomposition. Our second contribution is a tailored optimization strategy that identifies the walking model parameters, enabling the use of a walking model with sufficient fidelity that accurately describes complex pedestrian motion. We find the pedestrian motion that minimizes the difference between the measured strain and the predicted strain, leveraging the speed-up provided by the pROM and employing a gradient descent method with a modified basin-hopping scheme to quickly minimize this difference. We apply these methods to strain data collected from a real bridge and find that our preliminary results agree well with camera footage of the actual pedestrian motions.