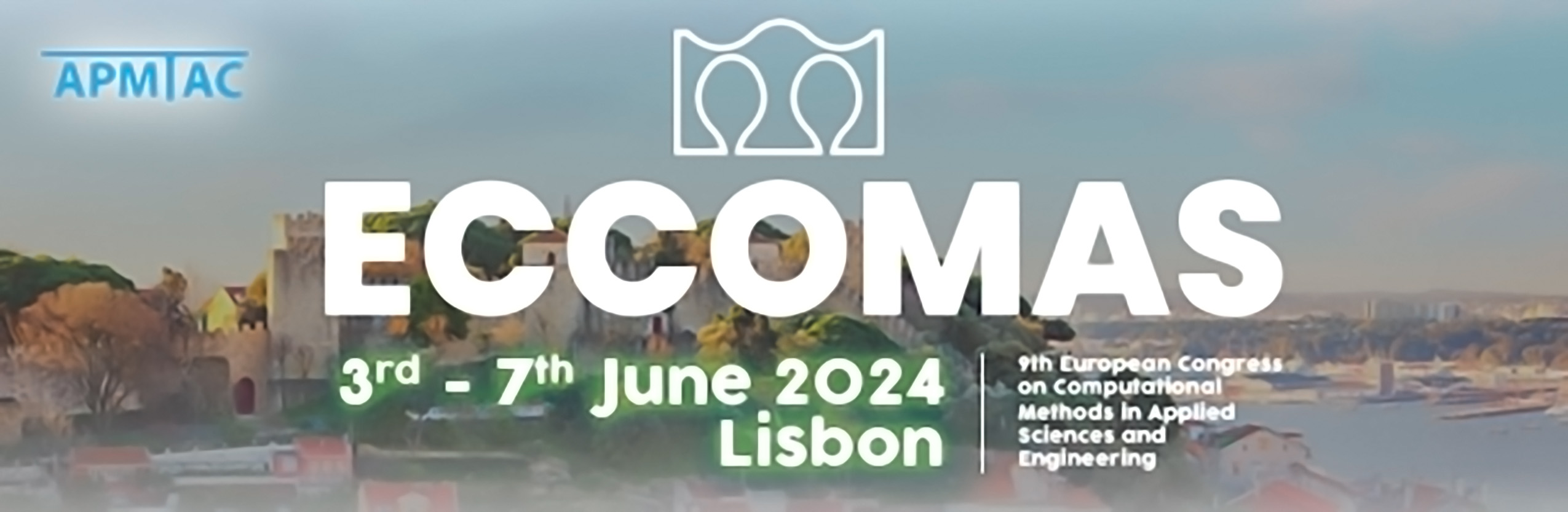
Towards Simulation Intelligence Testbeds: Enabling the industrial realisation of data-driven models and AI-first capabilities, and driving the evolution of computational engineering
Please login to view abstract download link
It is nothing new for the industrialisation of up-and-coming technologies, especially new computational approaches in legacy sectors of energy and manufacturing, to be stalled or killed due to an overwhelming conflux of industry-scale performance, integration, and reliability challenges: industrial controls & engineering workflows that are misaligned with (rather, misunderstood by) their new computational counterparts; engineering standards & safety margins that are disjoint or orthogonal with the performance metrics used in developing & verifying information-processing technologies; data-readiness and computing needs that are opaque to domain specialists & industry professionals, and mistakenly assumed “flight-ready” by those in R&D circles [1]. Machine learning and artificial intelligence (ML & AI) exacerbate these concerns, due to (1) amplified expectations based on premature or unvalidated proof-of-concepts, and (2) proliferation of uncertainties and failure-modes arising from data-driven modeling & simulation (M&S) workflows [2-4]. A rich area we choose to explore these concerns and potential solutions is ML-driven Computer Aided Engineering (CAE) in industrial sectors—notable instances include process optimization and inverse design in additive manufacturing based on probabilistic ML [5-6], inverse engineering advanced energy storage systems requiring prediction of multiphysics material properties that vary across multiple spatiotemporal scales [7,3], and more in complex industrial cyber-physical systems [8,9,3]. In this work, we propose several simulation intelligence (SI) [3] approaches with industrial cyber-physical systems (ICPS) workflows to resolve the above concerns, for which we derive information-theoretic measures of M&S reliability, and present experiments at multiple tech-maturity levels and data regimes [1]. The result is what we call “SI Testbeds”, which embody several critical improvements over industrial “Digital Twins”, and elucidate the co-evolving domains of AI/ML and CAE across industry sectors. (References in the submitted attachments)