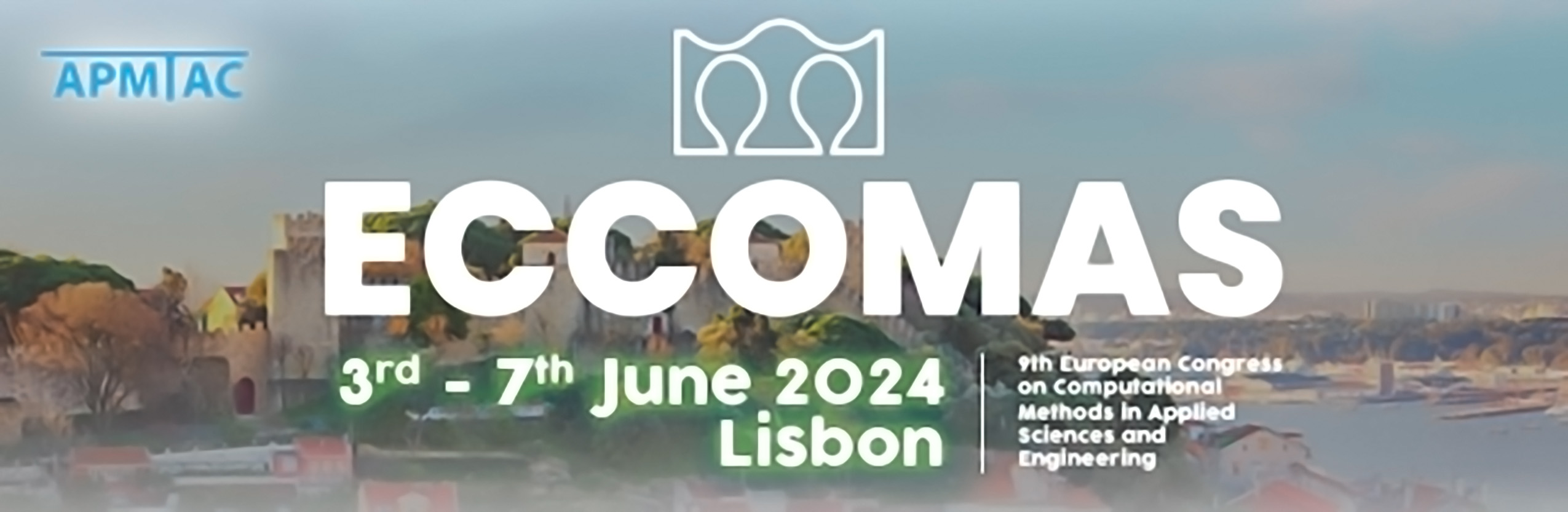
Materials Engineering using Generative Models and Multiscale Simulations
Please login to view abstract download link
Current CO2 emissions from concrete production are over 2 gigatons, mainly from cement production (60%) and fuel combustion in cement kilns (40%). By 2050, this is projected to cross 3 gigatons. From a materials’ perspective, sustainable concretes with new binders and renewable aggregates will significantly reduce CO2 emissions. However, due to the complexity of the physico-chemical processes that determine the micro-/mesostructure and properties of concrete and the additional layer of complexity involved in the devel-opment of novel sustainable ingredients, materials innovation is very challenging through purely experimental approaches. A synthesis of experimental approaches, physics-based multiscale models and machine learning (ML) can accelerate materials innovation and discovery. In this contribution, we present a novel computational framework for design- ing novel sustainable cementitious materials using generative models that are supported by multiscale physics-based computational modeling and simulation. The physics-based multiscale computational model is a reduced-order material modeling framework that combines geometrically implicit multi-level micromechanics and geometrically explicit mesoscale models. Data generated using this model is used to train a conditional vari- ational autoencoder that is conditioned on the required material performance indicators (compressive strength) and environmental impact factors. The material compositions designed by the generative model is deconstructed using physics-based multiscale simu- lations. The novel material compositions are finally evaluated and potential limitations with regard to manufacturability is discussed.