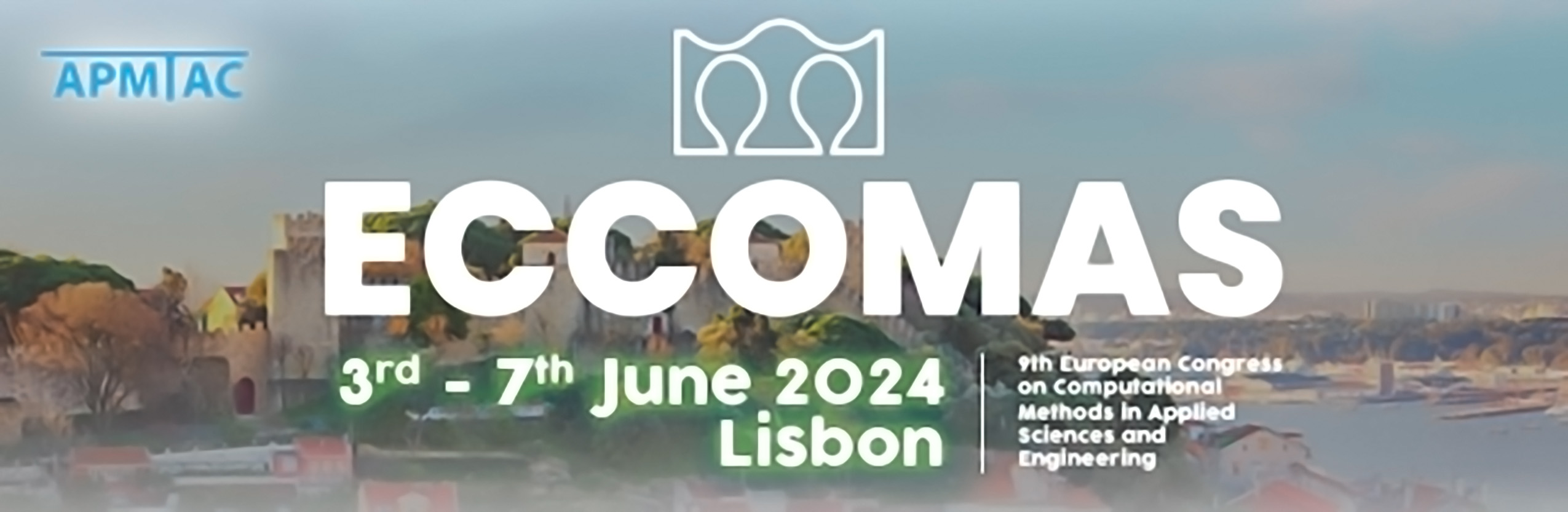
Data Driven Uncertainty Quantification with Neural Networks
Please login to view abstract download link
Using reduced order models we create a large amount of data in a reasonable time and train a neural network with this data to answer the following question: given this distribution of inputs what is the distribution of the quantity of interest? As an example we use an elastic block problem where the block is deformed by forces. The forces influencing the elastic block are uncertain and the elasticity of the block is also uncertain. Uncertainty quantification is usually done with some type of Monte Carlo sampling. This tends to be computationally expensive. The motivation to create machine learning models to address this problem comes from the ability of neural networks being computationally fast in solving PDE related problems. Accuracy of the estimates is not necessarily as good as the classical methods but the difference in computational cost is tremendous. In the context of digital twins a machine learning model which quickly estimates the effects of uncertainty could be useful. A digital twin of a complex system requires large computational resources. In order to do uncertainty quantification in the classical way of sampling one would have to run multiple of these digital twin simulations and compute quantities of interest from all of them. In some cases this may need an unfeasible amount of computational resources.