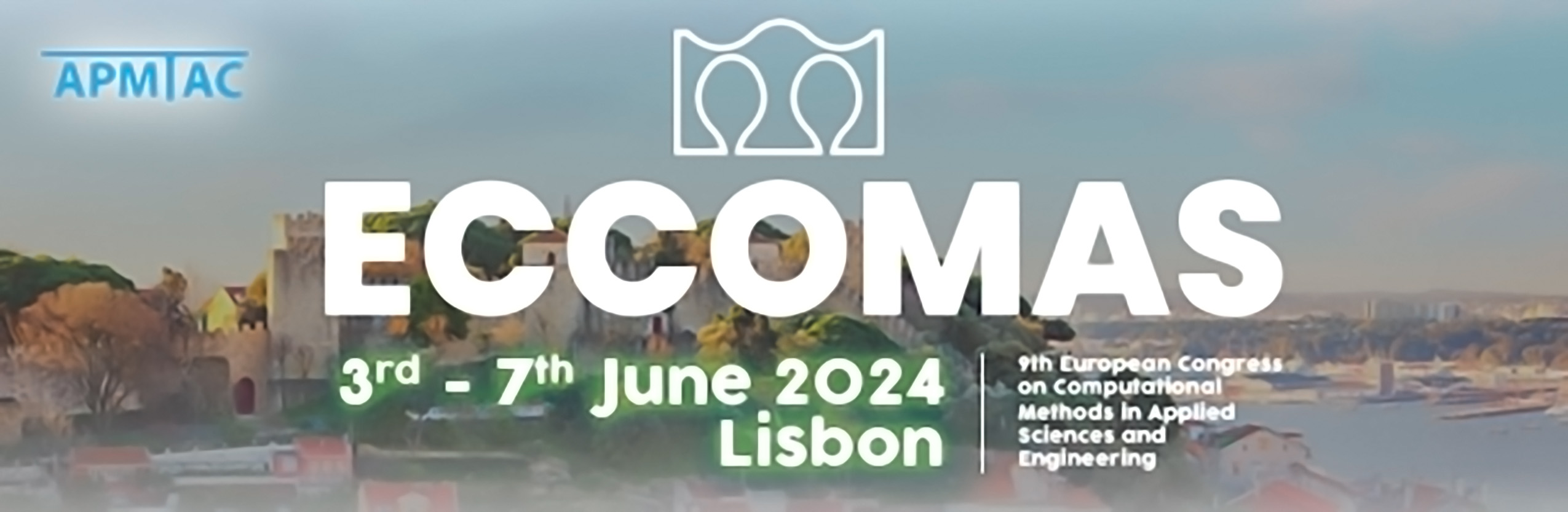
Bayesian Optimization for mechanical metamaterial design
Please login to view abstract download link
Mechanical metamaterials consisting of periodically arranged frames of beams and struts can offer superior and unprecedented property combinations, such as high stiffness and strength at low density, negative Poisson's ratios, or vanishing shear modulus. Traditionally, these materials have been designed with uniform thickness members to facilitate manufacturing and analytical modelling of their mechanical response. Consequently, the vast space of non-uniform designs has been under-explored. Without general analytical models, optimizing such novel architectures becomes highly challenging due to the expense of engineering simulations and physical experiments involved in the design process. In this work, we present a computational framework that leverages Bayesian optimization (BO) to guide the design of structured solids with optimal geometric parameters in a minimal number of simulations. The framework was applied to optimize the thickness profile of struts in a hexagonal honeycomb material for the uniaxial effective elastic stiffness and failure load due to plastic collapse or buckling. We additively manufactured and tested the best-found topologies at different relative densities (and prevalent failure modes), resulting in a 54\% increase in stiffness without compromising the buckling strength for slender architectures and a 63\% increase in elastic modulus and an 88\% increase in plastic strength for higher volume fractions \cite{kuszczak2023honeycomb}. Our results demonstrate an attractive, model-agnostic alternative to classical topology optimization techniques and highlight the potential of Bayesian optimization and solid material redistribution to enhance the performance of mechanical metamaterials.