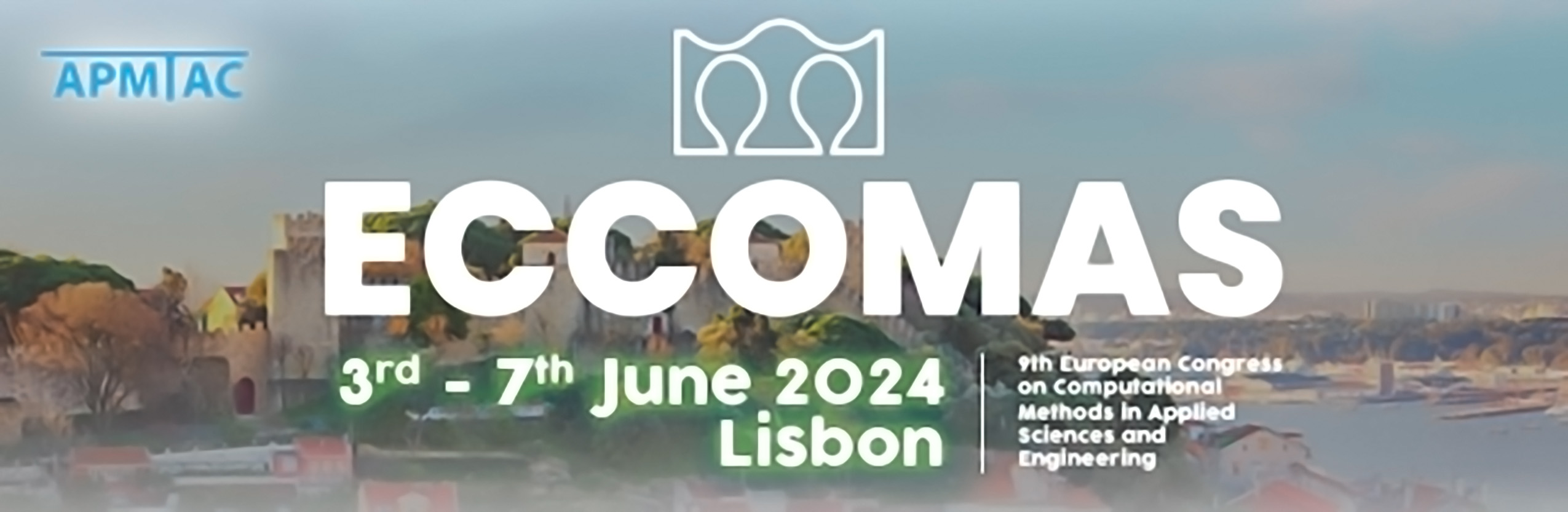
A Neural Network Strategy for the Modelling of Inelastic Solid Materials via Gradient-Free Optimisation
Please login to view abstract download link
A neural network-based surrogate model for inelastic solid materials simulations is presented. The network architecture incorporates the elastoplasticity equations and has been proven to be exact in one-dimensional elastoplasticity with hardening [1]. This strategy provides an alternative to the complex task of formulating constitutive equations for new materials due to the capability of learning directly from data. The strategy has a wide range of applications under the two main scenarios that generate the necessary data: (a) by performing physical experiments, and (b) by numerical homogenisation within the multiscale analysis. The network is constructed based on the concept of internal states and utilises only observable variables (strains and stresses), hence, a recurrent structure is required to account for the deformation history. Due to the challenging task of training recurrent networks, a supervised parallel optimisation (SPO) [2] framework has been developed, which combines the exploration and exploitation capabilities of one (or several) metaheuristic algorithms. In addition, a gradient-enhanced objective function along with the loss corresponding to the data fitting has been investigated and shown to deliver promising results. The trained network is embedded as a surrogate model into a finite element code on a Gauss point level, replacing a traditional library of algorithmic constitutive models. The stress update is obtained from the network output and the consistent tangent modulus is derived from the network architecture utilising the prescribed functional dependencies. Numerical experiments are presented using network surrogates trained with the Von Mises elastoplasticity model and a multiscale Gurson model for porous elastoplastic materials. REFERENCES [1] W. G. Dettmer, E. J. Muttio, R. Alhayki, and D. Perić. A Framework for Neural Network Based Constitutive Modelling of Inelastic Materials. Computer Methods in Applied Mechanics and Engineering, 420: 116672, 2024 https://doi.org/10.1016/j.cma.2023.116672. [2] E. J. Muttio, W. G. Dettmer, J. Clarke, D. Perić, Z. Ren, and L. Fletcher. A Supervised Parallel Optimisation Framework for Metaheuristic Algorithms. Swarm and Evolutionary Computation, 84: 101445. https://doi.org/10.1016/j.swevo.2023.101445.