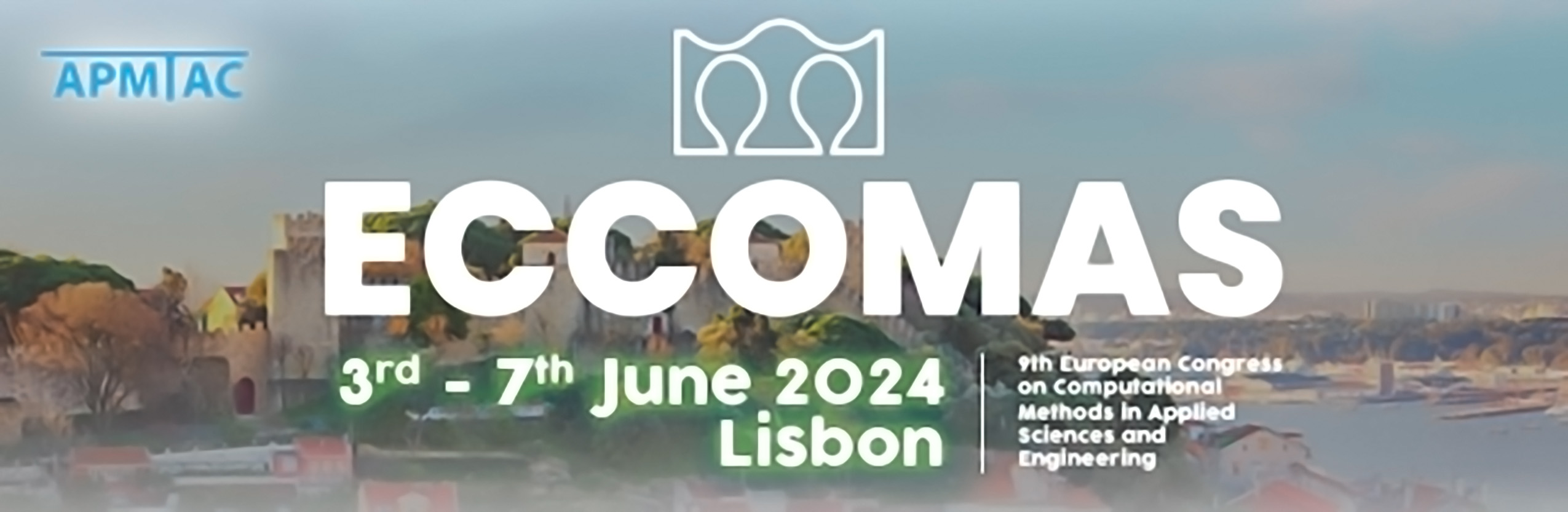
Deep learning-aided inverse design of porous metamaterials
Please login to view abstract download link
This study explores the generation of porous metamaterials through deep learning-based inverse design of open-cell foam. The dataset contains CT images of Representative Volume Elements (RVEs) at various deformation states, along with corresponding deformation-dependent porosity and anisotropic intrinsic permeability calculated using the lattice Boltzmann method (LBM). Our objective is to design structured metamaterials that mirror specified hydraulic properties, including porosity and permeability. To achieve this, we will utilize convolutional variational autoencoders (CVAEs), enabling us to generate complex metamaterial structures. A convolutional neural network (CNN) model is considered to train a hydraulic properties predictor. Subsequently, variational autoencoders (VAEs), with their ability to learn probabilistic mappings, will establish a relationship between input CT images and desired hydraulic properties. The encoder-decoder architecture of VAEs will allow to generate porous metamaterials that conform to specified porosity and permeability values. REFERENCES [1] Phu, N. T., Navrath, U., Heider, Y., Carmai, J., & Markert, B. (2023): Investigating the impact of deformation on foam permeability through CT scans and the Lattice-Boltzmann method. PAMM, e202300154. DOI: 10.100/pamm.202300154. [2] Zheng, L., Karapiperis, K., Kumar, S. and Kochmann., D.M. (2023): Unifying the design space and optimizing linear and nonlinear truss metamaterials by generative modeling. Nature Comm.. Vol. 14, pp. 7563. https://doi.org/10.1038/s41467-023-42068-x. [3] Aldakheel, F., Elsayed, ES., Zohdi, TI., Wriggers, P. (2023): Efficient multiscale modeling of heterogeneous materials using deep neural networks, Computational Mechanics 72, 155-171.