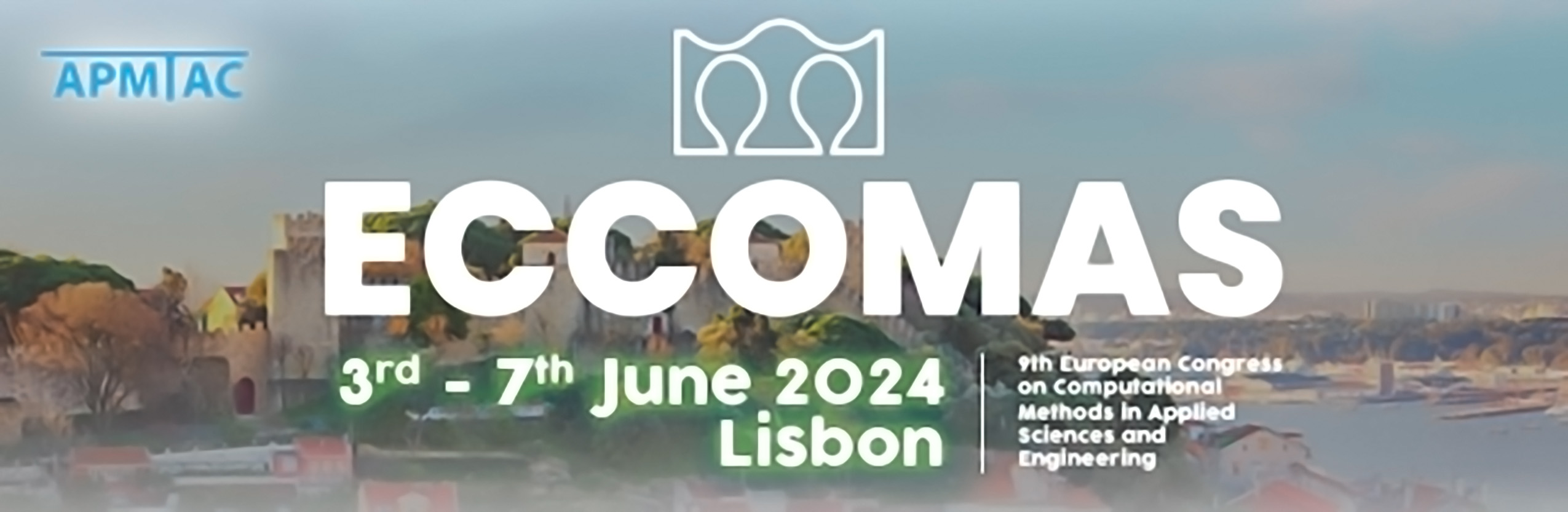
Trustworthy Scientific Machine Learning with SINDy and Ensemble Learning
Please login to view abstract download link
The sparse identification of nonlinear dynamics (SINDy) algorithm can identify dynamical system models purely from data. Compared to black-box machine learning methods, SINDy learns sparse ordinary (ODE) or partial differential equations (PDE) that are interpretable and generalizable, which is critical in achieving trustworthiness in scientific machine learning. Trustworthiness is particularly important when deploying machine learning models in high-consequence and safety-critical environments, where models need to be certifiable, e.g. by guaranteeing model stability, robustness, and generalization. A mathematical framework that provides tools for estimating these guarantees in the model discovery procedure is uncertainty quantification. In this work, we present an extension of SINDy using ensemble learning to perform computationally efficient uncertainty quantification. We show that ensemble SINDy can provide valid uncertainty quantification, and we show that it can perform correct variable selection with guaranteed convergence. We also discuss several challenges when applying SINDy to noisy experimental data sets, and present a range of methods to improve the effectiveness of SINDy, such as active learning, finding effective coordinates in which learned models are sparse, and automated hyperparameter tuning for model selection. Finally, we discuss how SINDy can be extended to identify parametric models, important in many applications where models need to be accurate over large parameter and operating regimes. Along with ensemble learning, these SINDy extensions enable the identification of accurate models that can provide robustness estimates and quantify the credibility of predictions, critical to make the learned models trustworthy. We demonstrate the applicability of SINDy and its extensions on a benchmark synthetic dataset and a challenging experimental dataset with relevance in adaptive structures and aeroelasticity.