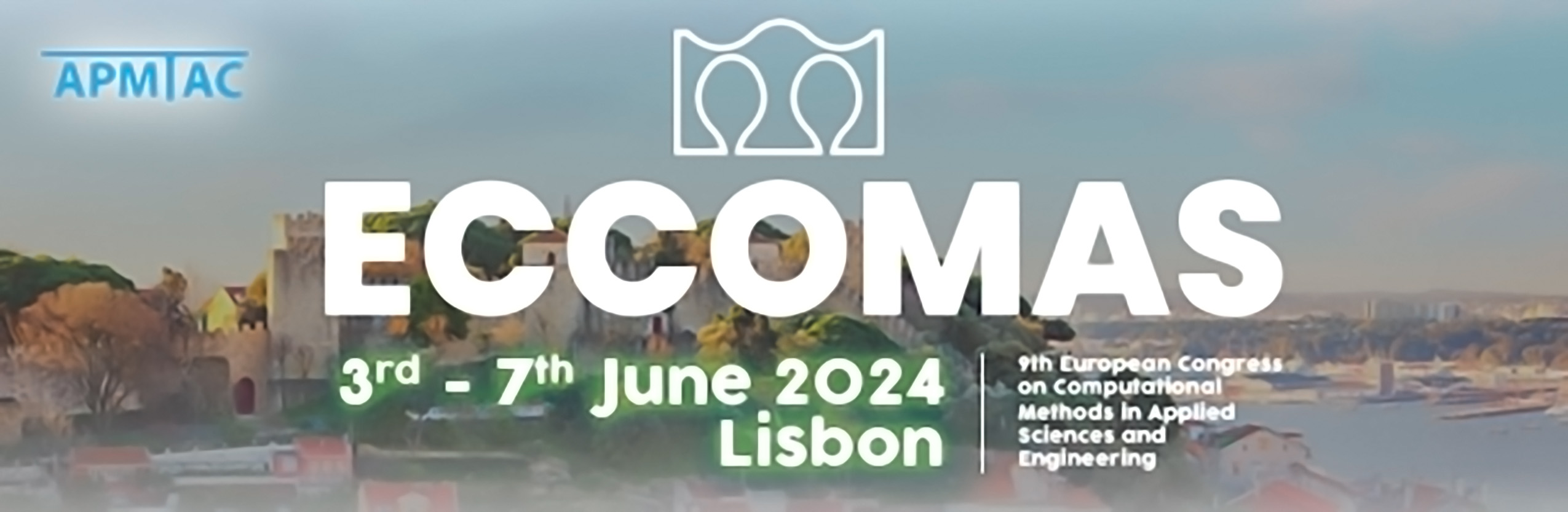
Evolving System Parameters: Set-Encoding for Online Nonlinear Dynamics Identification
Please login to view abstract download link
The promising outcomes of dynamical system identification techniques, such as SINDy [1], highlight their potential applications in diverse fields like manufacturing process monitoring and control. These methods provide distinct advantages in terms of qualitative interpretability and extrapolation, distinguishing them from "black box" approaches like Neural Networks. However, these techniques suffer from model updating in real-time, especially when the system parameters are likely to change during or between processes. Recently, the OASIS[2] framework introduced a data-driven technique to address the limitations of real-time model updating by training a feed forward neural network to perform sparse system identification, yielding interesting results. Nevertheless this model architecture fall short in capturing system dynamics, as it does not have a memory mechanism and it can not inherently capture the temporal dependencies in sequences. To improve upon the current methodology, we introduce set encoding for sequence data by using Deep-Sets[3] and Set-Transformers architectures. This approach ensures accurate adaptative model identification for complex dynamic systems sush as the Lorenz System, with variable input data size. Comparing our architecture to OASIS framework on different systems, we find that the set encoding architectures are more accurate for system identification based on historical data.