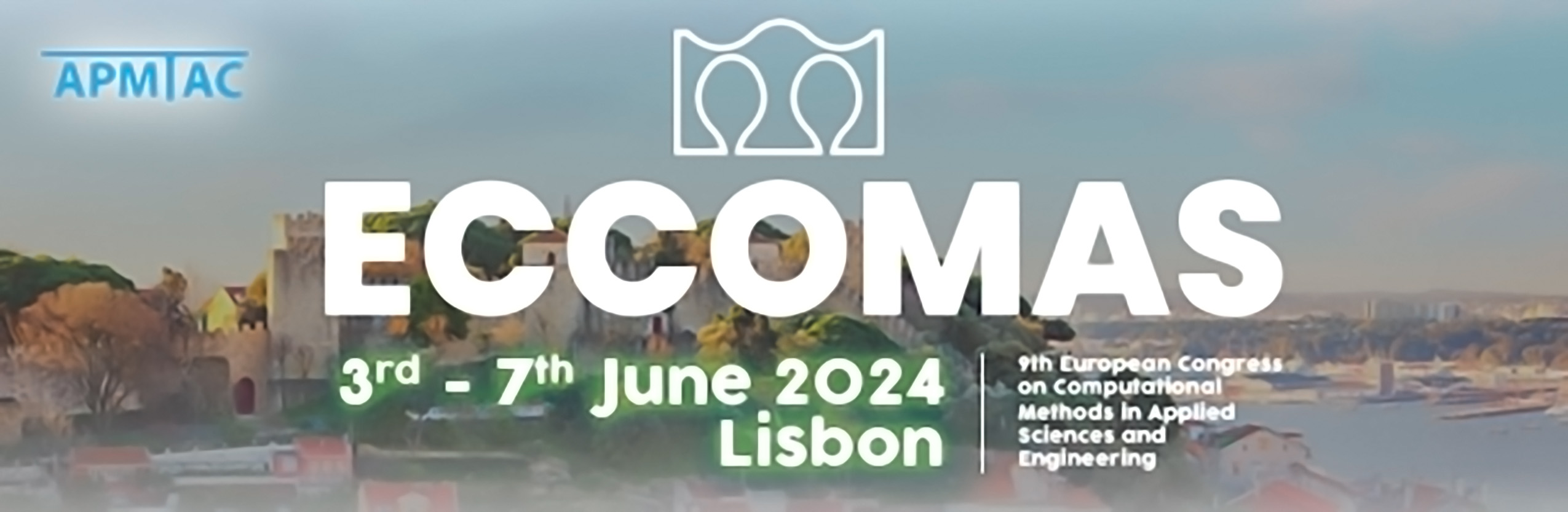
Structure-preserving deep learning
Please login to view abstract download link
Simulation technologies have become a useful tool to model many systems in a wide variety of disciplines, ranging from social to natural sciences. In the engineering context, the mathematical description of physical systems is crucial in order to have a deeper understanding of their future behaviour and take the best cost-effective decisions in terms of design optimization. In the last decades, with the irruption of the so-called fourth paradigm of science, a growing interest is detected in the machine learning of these scientific laws. Many of such approaches completely discard centuries of scientific knowledge in favour of pure data-driven models, whose application in real industry remains unclear over traditional mathematically-founded methods. This work aims to develop deep learning methodologies to learn dynamical systems from data. The scientific knowledge is incorporated into the model with the imposition of the correct thermodynamical structure of the problem. Thus, it is a hybrid approach between data-driven black-box methods and traditional analytical formulations. First, we develop structure-preserving deep learning methods by using several inductive biases, which enforce the metriplectic structure of dynamics by construction and exploit the domain structure using the geometric deep learning principles. These state-of-the-art neural network architectures enable the identification of the dynamics of unknown systems even with highly nonlinear behaviour. Additionally, the resulting integration scheme achieves both fast and accurate predictions with low computational power and memory storage requirements. Last, we show the performance of this technology with an augmented reality demo for the interaction of deformable solids in real-time.