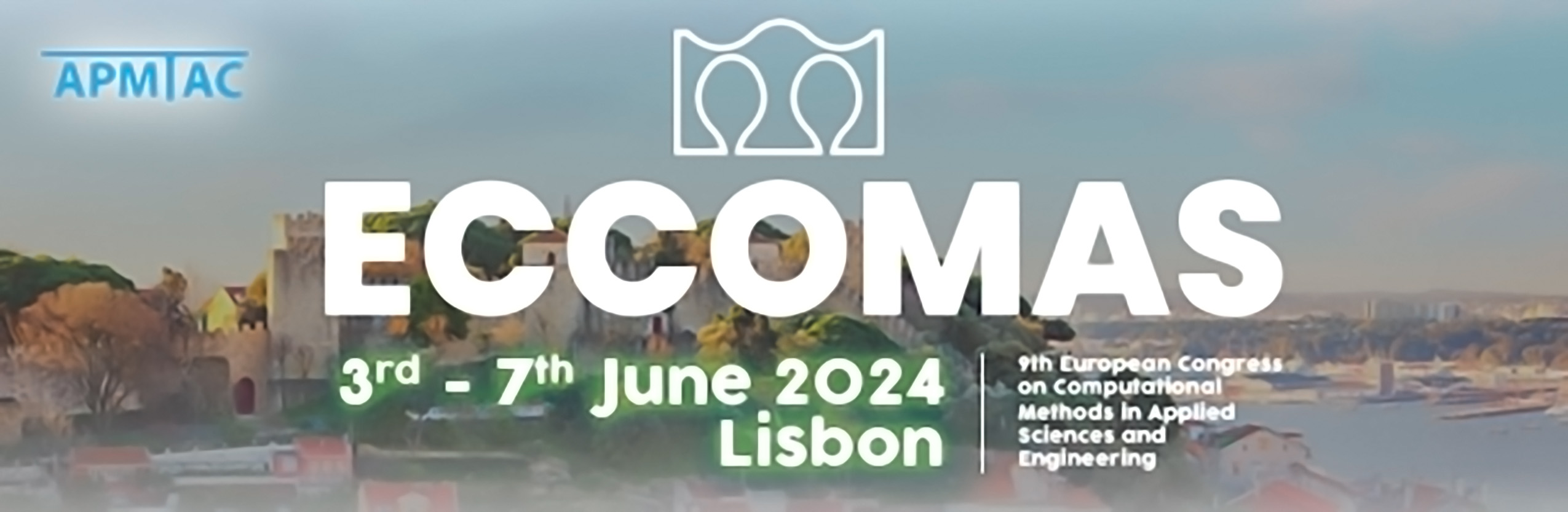
Real-Time Simulation of Plastic Deformation Using Long Short-Term Memory Multi-Task Neural Networks
Please login to view abstract download link
Accurate modeling of deformation in structures is essential in a range of applications, such as minimally invasive therapies and surgical robotics. However, computational challenges persist due to material nonlinearity and complex geometries. In this work, we investigate a surrogate modeling framework using neural networks for the real-time simulation of medical devices, with a focus on catheters. We employ proper orthogonal decomposition (POD) for efficient data compression, ensuring the model receives and processes only the most essential information. Long short-term memory (LSTM) networks are utilized for their proficiency in capturing temporal dependencies, crucial for predicting the dynamic behavior of a structure [1]. Multi-task learning (MTL) is incorporated to enable a single neural network to simultaneously learn various related tasks such as displacement, stress, and strain. This integration enhances the model's precision and broadens its applicability [2]. These methodologies aid in effectively simulating a simplified catheter, which demonstrates elastoplasticity and kinematic hardening, initially tackled as a cantilever problem. Our model demonstrates the ability to capture complex nonlinear material behaviors, showing a improvement in generalization capabilities over single-task models. The core of our analysis lies in comparing the performance and adaptability of multi-task versus single-task neural networks, focusing on their efficiency and accuracy in handling complex cases. This research aims to advance the field of real-time deformation modeling, contributing to the development of computational methods in both engineering and medical domains.