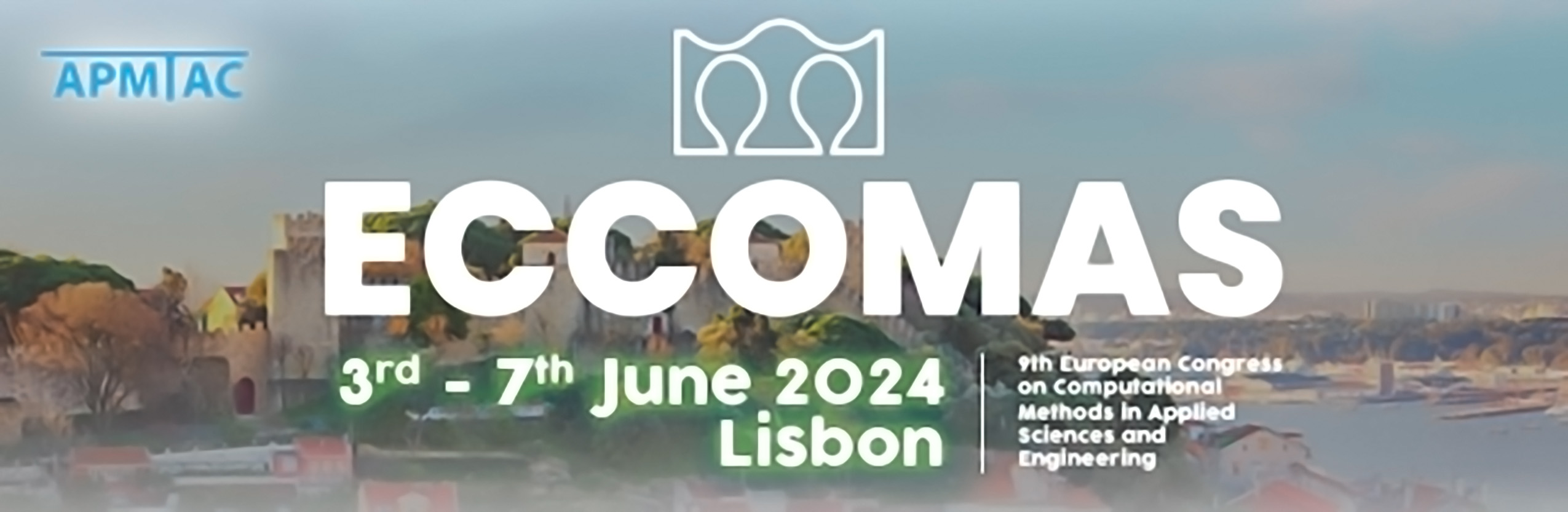
Optimizing Physics-Informed Neural Networks for Pulsatile Non-Newtonian Channel Flows
Please login to view abstract download link
We investigate the flow of non-Newtonian fluids, prevalent in various industrial and medical applications such as blood flow in arteries and the transport of chemical solutions using oscillatory pumps, specifically focusing on pulsatile shear-thinning flows through a straight channel. Traditional approaches have been used in previous studies, but recently, physics-informed neural networks (PINNs) have emerged as a novel method for solving systems of partial differential equations in such situations. PINNs have been applied to solve forward and inverse problems arising from steady-state flows of power-law fluids in various geometries, and to model channel flows of fluids with different rheological constitutive models under varying flow protocols. In this study, we apply PINNs to solve pulsatile shear-thinning flows in a two-dimensional channel. We explore models with different boundary conditions, network sizes, numbers of training points, activation functions, and loss weights using case studies complemented by Gaussian-processes-based Bayesian optimization. Our results suggest that increasing the number of training points is more beneficial than expanding the network size for our problem of interest. Furthermore, the use of swish activation, hard-REQ constraints for boundary condition enforcement, and assigning large loss weights for the continuity loss term enhance the performance of the model. The optimized model aligns well with the reference numerical solution, indicating that appropriately trained PINNs can be utilized as a method for simulating transient shear-thinning flows.