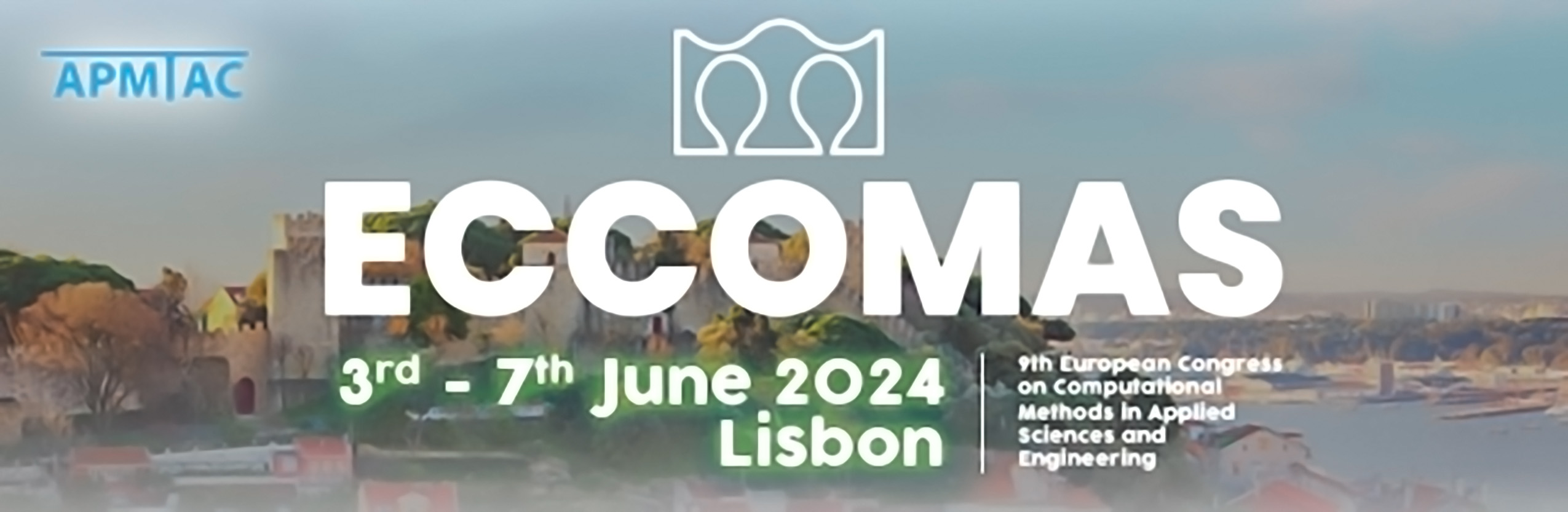
A Neural Network Approach to Learn Delay Differential Equations via Pseudospectral Collocation
Please login to view abstract download link
The use of neural networks to learn the dynamics of Delay Differential Equations (DDEs) has been recently considered in [1]. The methodology proposed therein relies on reducing to finite dimension the infinite-dimensional dynamical system associated to the DDE by following the approach presented in [2]. In particular, an approximating Ordinary Differential Equation (ODE) is obtained by using a discretization based on the standard Euler’s method. In this talk we illustrate a recent extension relying on pseudospectral collocation, which leads in general to a more efficient approximation of the underlying DDE. We focus our interest on analyzing the role of the degree of the collocation polynomial in terms of the effectiveness of the learning algorithm and in view of understanding its interplay with the network hyperparameters. Experimental results refer both to the celebrated Mackey-Glass equation as well as to a delay model for supply chains. REFERENCES [1] X. Ji and G. Orosz, Learning Time Delay Systems with Neural Ordinary Differential Equations, IFAC PapersOnLine 55(36):79-84, 2022. [2] D. Breda, O. Diekmann, M. Gyllenberg, F. Scarabel and R. Vermiglio, Pseudospectral discretization of nonlinear delay equations: new prospects for numerical bifurcation analysis, SIAM J. Appl. Dyn. Sys. 15(1):1-23, 2016.