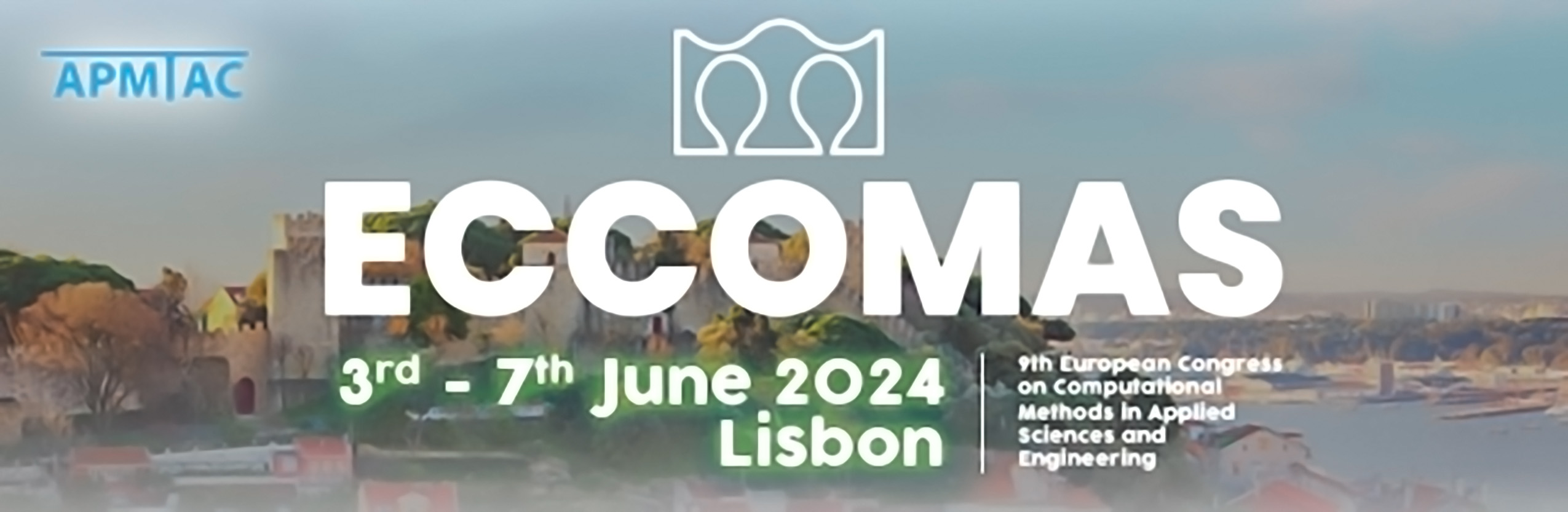
A deep learning approach for the reconstuction of 3D geometries and fields
Please login to view abstract download link
In many engineering applications, local sensors provide only a partial view of a phenomenon's geometry and physical fields. Ensuring a high-fidelity reconstruction of both is key for developing accurate data-model integration and statistical learning methods, especially in scenarios where no additional data acquisition modalities are available. In this talk, we present a novel approach that employs neural networks (NNs) to reconstruct a 3D object's geometry and distributed spatio-temporal quantities of interest. A first model is optimized for learning signed distance functions that generate implicit representations of 3D shapes. For its training process, we design loss functions that employ pointwise data in an unsupervised or semi-supervised manner and include suitable geometric regularization terms. A second model is then optimized to predict spatio-temporal fields over the reconstructed geometry. By incorporating spatial constraints given by the implicit representation of the first NN in the training process of this second NN, we guarantee coherence with the actual underlying phenomenon. We present two numerical experiments validating the proposed deep learning approach on an idealized dataset sampled from a sphere and on an electroanatomical dataset. The latter consists of noisy and sparse geometric points and associated electrograms measured by a catheter and is employed to guide electrophysiologists in navigating heart chambers' geometry and electrophysiological properties. Numerical results show that our NN models generate an accurate and differentiable 3D reconstruction of the left atrium and its electrophysiological fields.