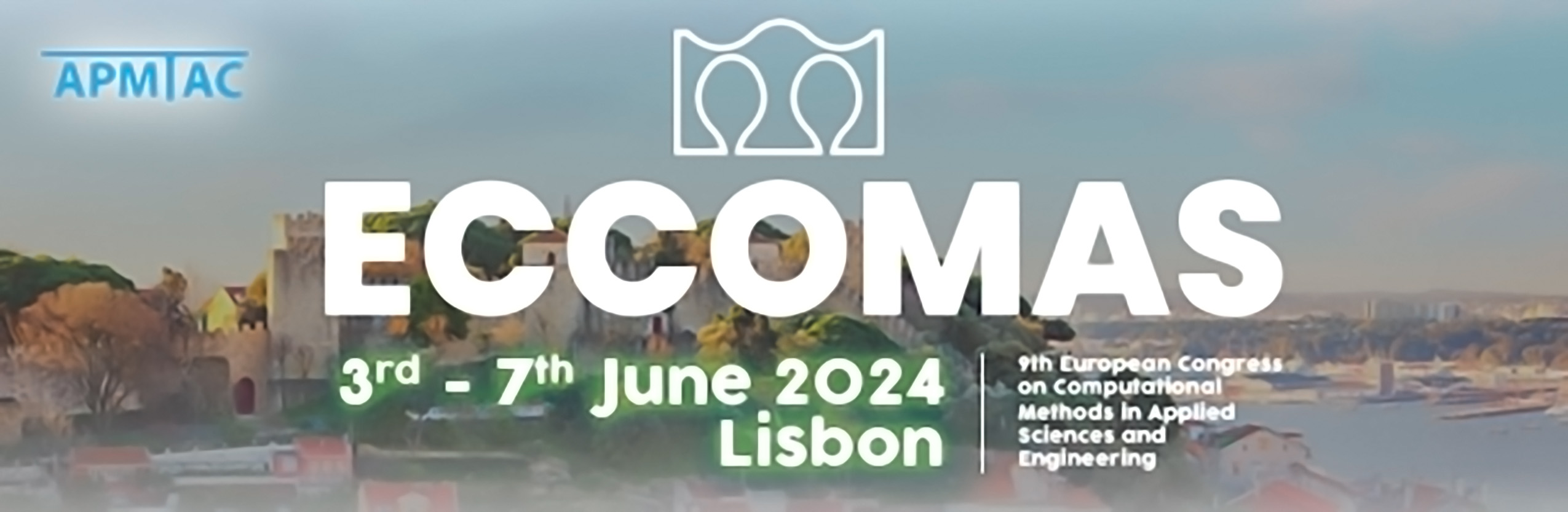
Co-design Optimization of Adaptive Structures using Deep Reinforcement Learning
Please login to view abstract download link
Rapid advances in material science and additive manufacturing are enabling a new class of adaptive autonomous systems, such as morphing wing drones and soft robotic swimmers. These systems can adapt their shape to uncertain and rapidly changing flow environments and are likely to provide superior performance compared to conventional rigid systems, as demonstrated by the incredible performance of birds and insects that exploit the flexibility of their wings to perform agile and efficient flight maneuvers. Several challenges remain in understanding how the adaptive structure of the autonomous system interacts with unsteady flows, how the structure has to be designed and optimized to achieve system- level performance gains, and how real-time control of the high-dimensional, nonlinear fluid-structure dynamics is achieved. In particular, the intertwined processes of optimizing the design and learning a control policy of adaptive structures present a great challenge. In this work, we present ideas around co-design optimization of adaptive structures using Deep Reinforcement Learning (DRL), which has been successfully applied to robotics and energy system design and control. Moreover, DRL has been applied to active flow control problems and has shown significant promise in learning sophisticated control policies that interact in environments with high-dimensional and nonlinear unsteady flows. In our co-design approach, the physical design of the system and the active flow control policy are simultaneously optimized. This allows a better exploration of the interactions and a better exploitation of the interdependencies between the design and control of the adaptive structure operating in unsteady flows. We show that co-designing adaptive structures improves system-level performance compared to sequentially optimizing design and control. We demonstrate our co-design approach on several benchmark continuous control environments and challenging fluid-structure interaction problems, highlighting the benefits of co-design optimization applied to adaptive structures.