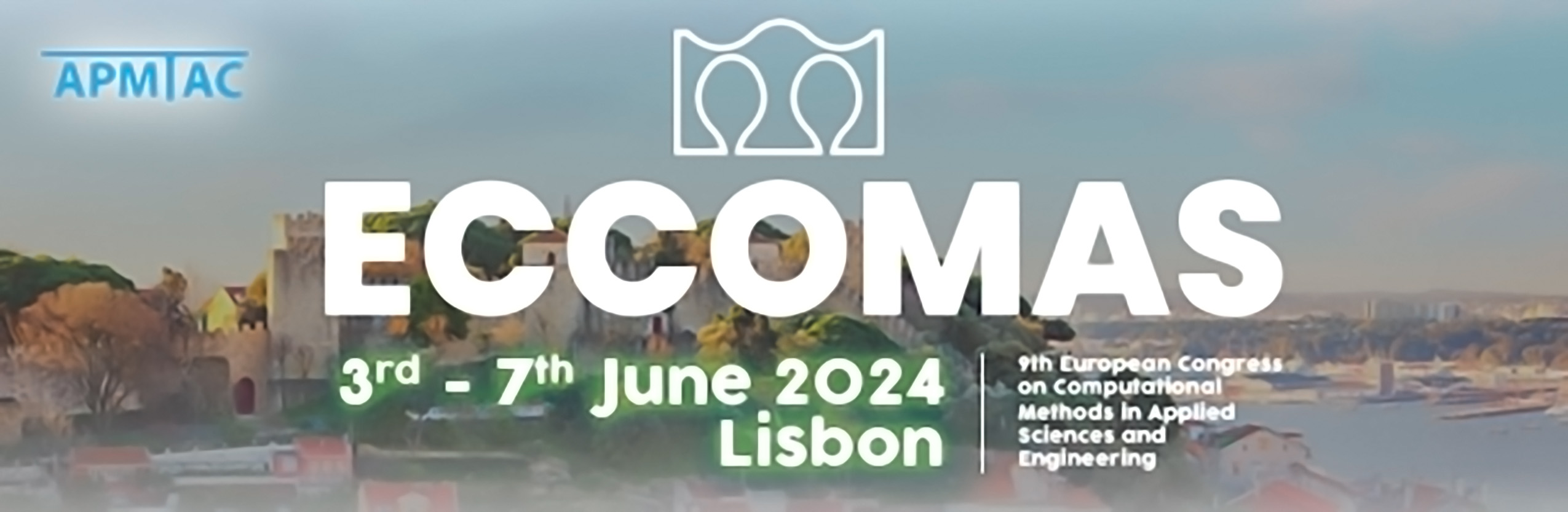
Challenges in Training Generative Models for Kirigami Metamaterials
Please login to view abstract download link
Generative machine learning models have revolutionized image generation in recent years. Their extension to other tasks, such as the generation of mechanical metamaterials, seems to proceed smoothly [1]. Yet, this perceived success could be partly attributed to survivorship bias. Indeed, a large portion of published works focuses on certain families of mechanical metamaterials with continuous design spaces, often overlooking those with complex design constraints that need to be learned. To explore when modern generative models struggle to generate suitable samples and understand why this happens, we examine a class of kirigami metamaterials -- planar sheets with straight cuts. In kirigami metamaterials, intersections between cuts are typically avoided to prevent disconnected regions, resulting in complex design restrictions [2]. In this study, we test the capabilities of the four most popular generative models -- the Variational Autoencoder (VAE), the Generative Adversarial Network (GAN), the Wasserstein GAN (WGAN), and the Denoising Diffusion Probabilistic Model (DDPM) -- in generating kirigami structures without intersections. We demonstrate that prohibiting intersections can prevent the identification of a suitable similarity measure between samples. Furthermore, we show that VAE and WGAN, by definition, rely on classical Euclidean distance -- a metric ill-suited for the considered metamaterials. This is the primary reason for their subpar performance in generation and their inability to learn complex relationships between cuts. Such insights highlight a significant limitation in using modern generative models for the design of diverse mechanical metamaterials. Given this, we find the investigated kirigami metamaterials to be a valuable benchmark for the future development of relevant generative algorithms. [1] X. Zheng, X. Zhang, T. Xubo and I. Watanabe, Deep Learning in Mechanical Meta- materials: From Prediction and Generation to Inverse Design. Advanced Materials, vol. 35, p. 2302530, 2023. [2] J. Grima, L. Mizzi, K. Azzopardi and R. Gatt, Auxetic Perforated Mechanical Meta- materials with Randomly Oriented Cuts. Advanced Materials, vol. 28, pp. 385–389, 2016