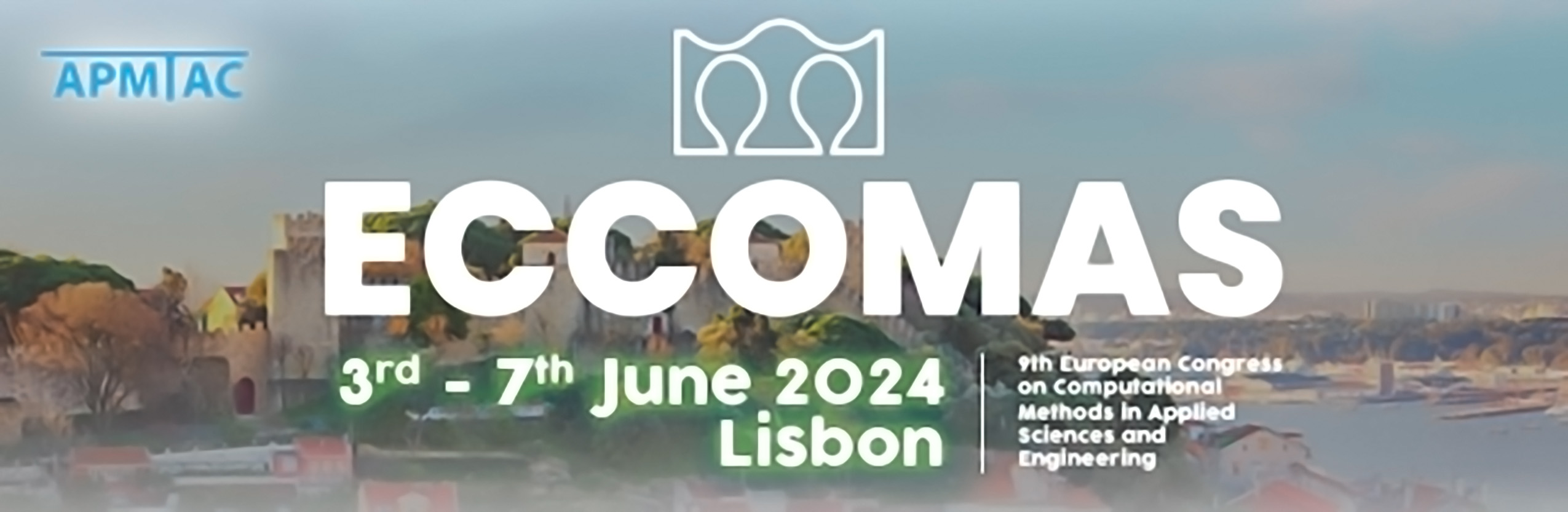
Efficient Bayesian Inference for Parametric PDE Inverse Problems using ZigZag Sampling and Approximate Gradients
Please login to view abstract download link
A key challenge in structural health monitoring is the inference of material properties from measurements. The challenge is particularly acute for cases that involve a spatial distribution of uncertain material parameters. These spatially distributed parameters form a random field with high stochastic dimensionality. Bayesian analysis offers a robust method to infer material parameter distributions. However, its dependence on Markov chain Monte Carlo (MCMC) approximations, which require many model evaluations, limits the applicability of Bayesian analysis, especially when the model, e.g. a finite element model, is expensive to evaluate. To address this challenge, we investigate the applicability of continuous-time sampling algorithms based on piecewise deterministic Markov processes (PMDP) and, more specifically, the ZigZag sampler for efficient exploration of the posterior in high-dimensional parameter spaces. In contrast to first order MCMC algorithms such as Hamiltonian Monte Carlo, the simulation of a PDMP requires access to not only the gradient of the log-joint of parameters and observation data but also a global upper bound thereof. The tighter the bound, the more efficient the sampling. These quantities are available for some canonical distributions but are difficult to obtain in most practical settings. We investigate two strategies to simulate PDMPs approximately: 1) Train a surrogate model to compute the gradient and its upper bound globally 2) violate the requirement for a global upper bound and find a good local approximation of the required quantities. In both scenarios, we introduce a bias but can obtain unbiased samples from the posterior by combining the approximate ZigZag sampler with a non-reversible Metropolis step. We showcase the efficacy of the two variants of the proposed algorithm through its application to inverse problems involving finite element solvers for parametric PDEs. We explore how well the algorithm's reported excellent mixing and low autocorrelation in high-dimensional parameter spaces translate to engineering applications. We present a strategy to mitigate this bias introduced by the approximation and report on the trade-off between statistical accuracy and computational efficiency. Furthermore, we compare our algorithm with related strategies to overcome the implementation challenges of sampling methods based on PDMPs.