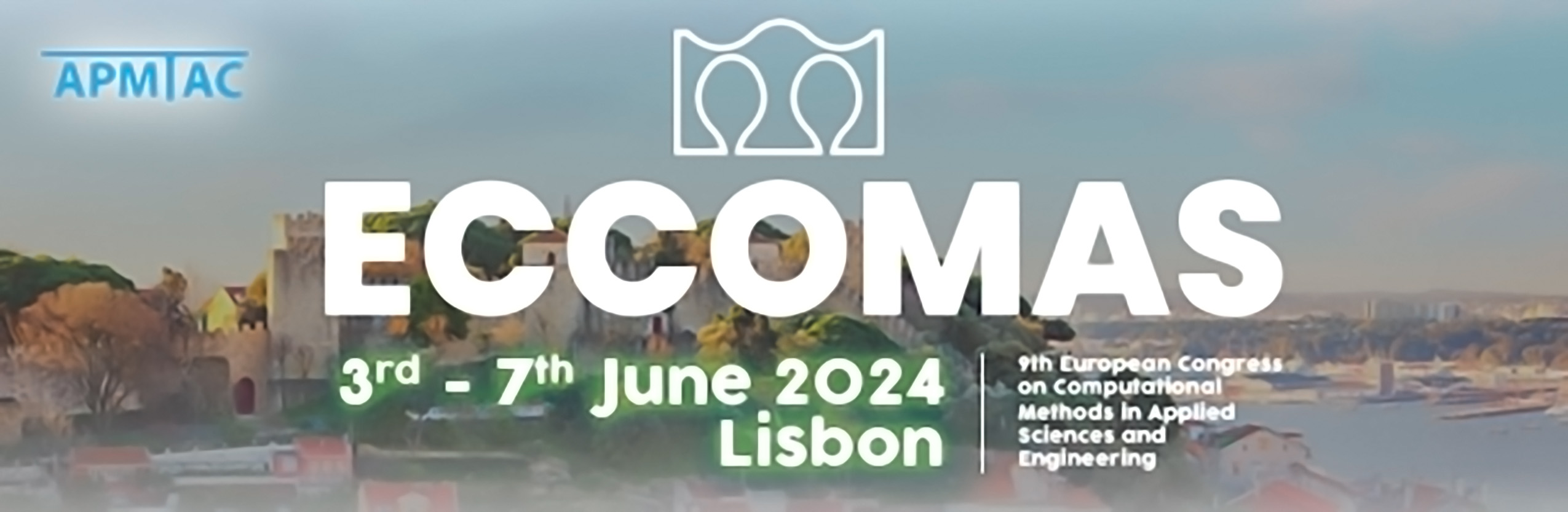
Variational Physics-Informed Neural Networks for Non-smooth Differential Equations in Solid Mechanics
Please login to view abstract download link
Physics-Informed Neural Networks (PINNs) have shown their capabilities in solving both forward and inverse problems across a variety of fields. A neural network approximates the unknown variables such that the strong form, alongside given initial and boundary conditions, is fulfilled. This framework has been extended in prior works, adopting the variational form into the loss function, leading to Variational Physics-Informed Neural Networks (VPINNs). The present contribution specifically focuses on the application of VPINNs for non-smooth partial differential equations. In contrast to PINNs, a mesh-based method is employed, simplifying geometry description by leveraging a standard finite element mesher. This approach enhances flexibility, particularly in handling complex domains. Deriving the variational form by applying test functions and, crucially, integration by parts, reduces the order of the differential operator. The nature of this weak form requires a lower regularity of the approximation. As an alternative to the conventional refinement of collacation points, this approach allows h- and p-refinement leading to high accuracy particularly in solving PDEs with steep solutions. The role of test functions, integration scheme, and element distribution is investigated in detail. We demonstrate the results on 2D solid mechanics problems.