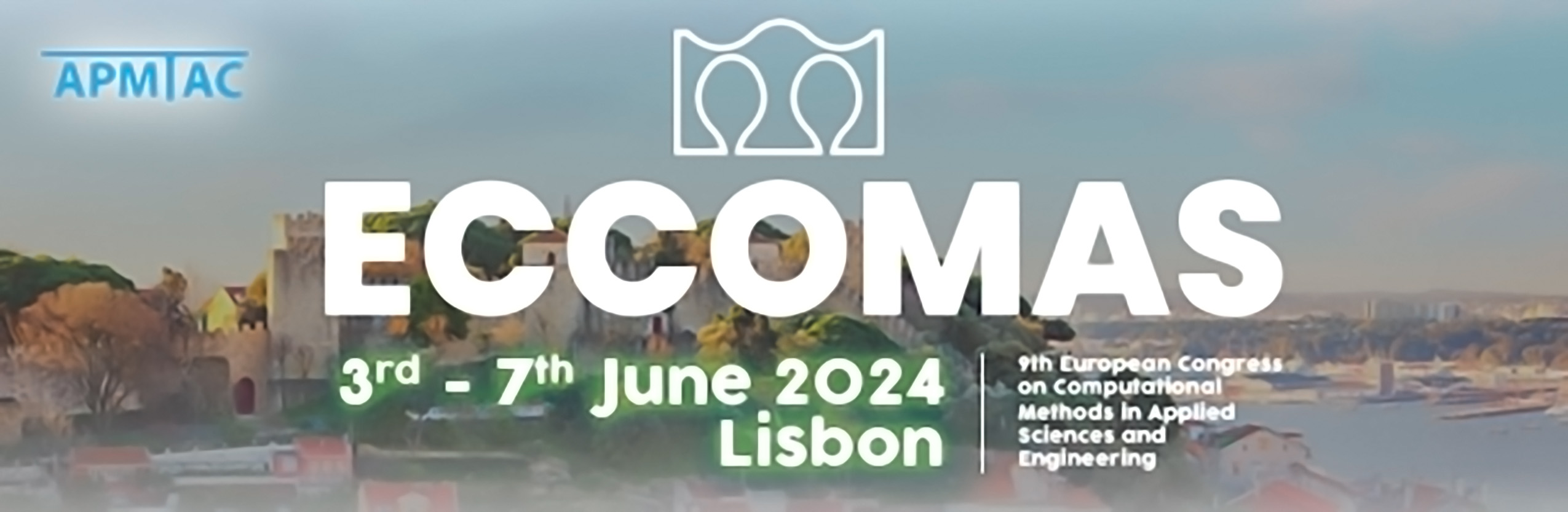
A data-driven learned discretization approach in finite volume schemes for compressible fluid dynamics
Please login to view abstract download link
The recent development of Machine Learning (ML) and Deep Learning methods, coupled with recent advances in GPU-based computing defined new promising techniques for the numerical resolution of PDEs entirely solved with ML, as well as tuning existing algorithms like learned corrections or discretizations. This research uses the coupling of finite volume numerical schemes and neural networks to learn the discretization of the spatial derivatives of partial differential equations (PDEs) in order to better resolve the small spatial scales. We use approximate solutions of the 1D and 2D Euler equations obtained on a fine Cartesian grid for the reference database in order to learn an optimal spatial discretization on a coarse grid, even with discontinuities in the solution. This is often described as super-resolution. We post-process the outputs of the neural network to guarantee consistency of the space discretization, and penalty functions are added for regularization. As a result, we show that the learned spatial discretization is independent of the time discretization and also independent of the grid on which the solution is calculated. Different types of boundary conditions are included in the database and learned by the neural network. For the latter, numerical experiments on solutions with shocks in one and two space dimensions are proposed to evaluate the performances of the present approach. We will show some preliminary results on the generalisation of the method on a unstructured grid using Graph Neural networks (GNN) and taking boundary conditions into account. We find that our method outperforms the existing reference finite volume scheme, allowing us to integrate twice as precisely on a same grid.