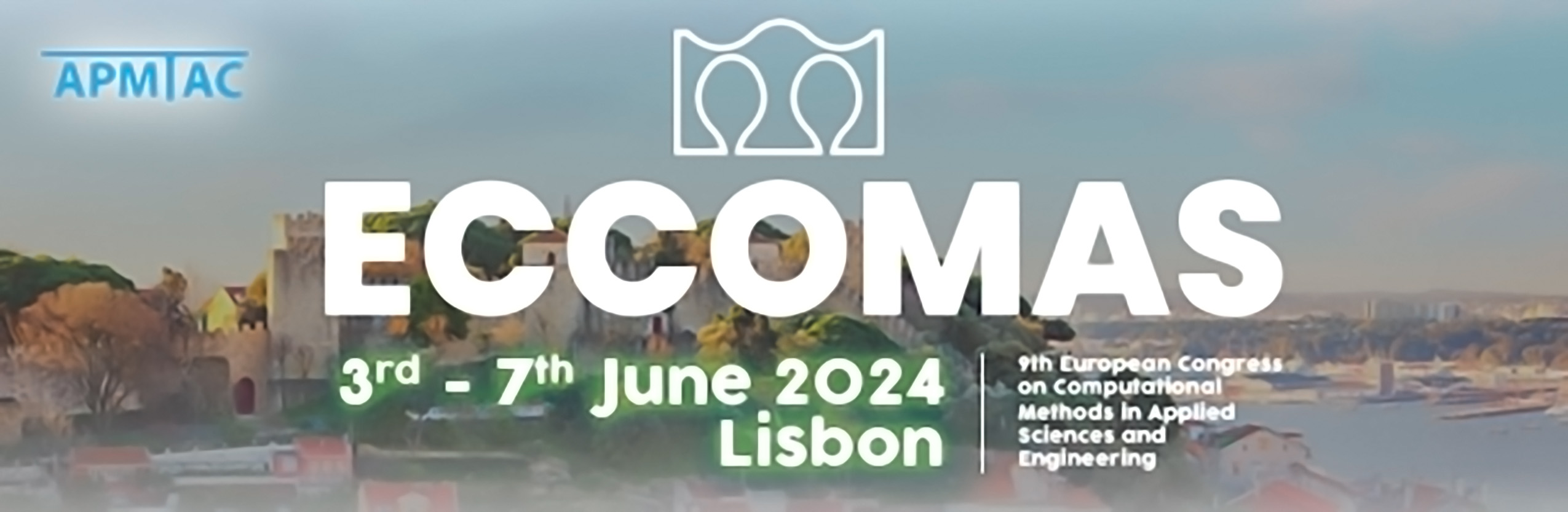
Damage Diagnosis of Buildings via BI-LSTM and GRU Deep Learning Models with Attention Mechanism
Please login to view abstract download link
Long Short-Term Memory (LSTM) and Gated Recurrent Unit (GRU), originally designed for time series data, have proven successful in various applications. The self-attention mechanism, a pivotal component of the Transformer model, empowers AI models to selectively concentrate on crucial segments of input data while mitigating noise and filtering out irrelevant information.This research specifically concentrates on the integration of AI deep learning models, such as BI-LSTM, GRU, LSTM with attention, and GRU with attention. The goal is to develop state-of-the-art deep learning models for structural response modeling and damage diagnosis under earthquake conditions of buildings. A numerical model of a seven-storey shear frame structure, subjected to 26 historical earthquake events as ground excitation, is employed to generate structural response data for both training and testing purposes. Structural damage was simulated by modifying the column sections. Three distinct structural damage scenarios were developed: non-damage (healthy state), single-story damage, and double-story damage. All relevant data were from nonlinear seismic dynamic analysis, which was subsequently divided into training and testing datasets. The training dataset comprised responses from undamaged buildings, establishing a baseline for healthy structures. In contrast, the testing dataset included responses from structures exposed to varying degrees of damage. The AI models underwent training using non-damaged state data to assess the prediction accuracy of four distinct deep learning models and establish a baseline for healthy structures. Subsequently, the damage state data were employed to evaluate prediction errors and determine the feasibility of identifying the damaged story. Research findings indicate that BI-LSTM and GRU exhibit effective predictive capabilities for structural responses, demonstrating both feasibility and accuracy. The incorporation of the attention mechanism proves highly beneficial, resulting in a notable reduction in network prediction errors and an enhancement in judgment accuracy.Through the amalgamation of the established health standard line with the prediction error broken line for the current structure, aiding in the identification of the structural damaged condition and pinpointing specific damaged locations.